The future of personalized healthcare: Predictive analytics
For full access to this report and all of our research, become a Rock Health partner—email partnerships@rockhealth.com.
We distinctly remember the moment that scientists claimed victory against all nature of future disease after the human genome had successfully been decoded. However, over the ensuing decade-plus, it has become clear that our health is not quite that deterministic. Clinicians must weigh not just a string of nucleotides when making decisions about our care, but must also incorporate a growing set of health data that is generated and controlled by patients. Incorporating this data into health care to enable better decisions is at the heart of this report. The benefits of using predictive analytics are the same as many categories of digital health: better care and lower costs. The difference is that the path to realizing these benefits—through personalized care—is only possible by implementing these technologies. The concern that care will be reduced to a set of algorithmically-derived probabilities is important and real. But the promise is as well.
Background
When the human genome was completely sequenced back in 2000, there was so much hope and excitement around the ability to personalize medicine, or drugs, to an individual’s genomic data.
[Genome science] will revolutionize the diagnosis, prevention, and treatment of most, if not all human diseases.
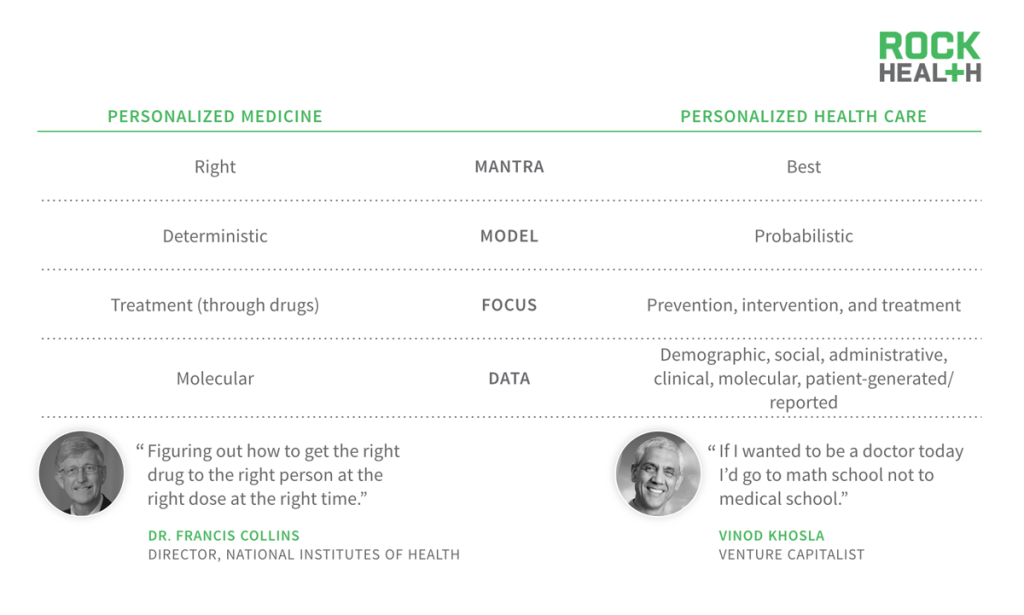
Landscape
Predictive analytics is not reinventing the wheel. It’s applying what doctors have been doing on a larger scale. What’s changed is our ability to better measure, aggregate, and make sense of previously hard-to-obtain or non-existent behavioral, psychosocial, and biometric data.
Combining these new datasets with the existing sciences of epidemiology and clinical medicine allows us to accelerate progress in understanding the relationships between external factors and human biology—ultimately resulting in enhanced reengineering of clinical pathways and truly personalized care.
Predictive analytics is the process of learning from historical data in order to make predictions about the future (or any unknown).
For health care, predictive analytics will enable the best decisions to be made, allowing for care to be personalized to each individual.
Our report focuses on how predictive analytics is directly impacting patient care.
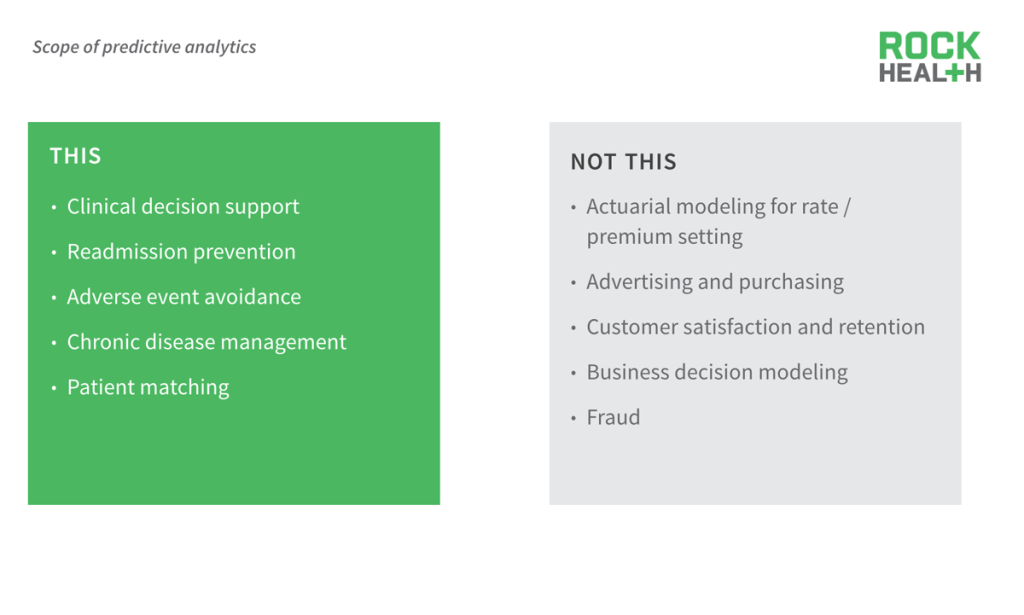
The goal of predictive analytics in any field is to reliably predict the unknown.
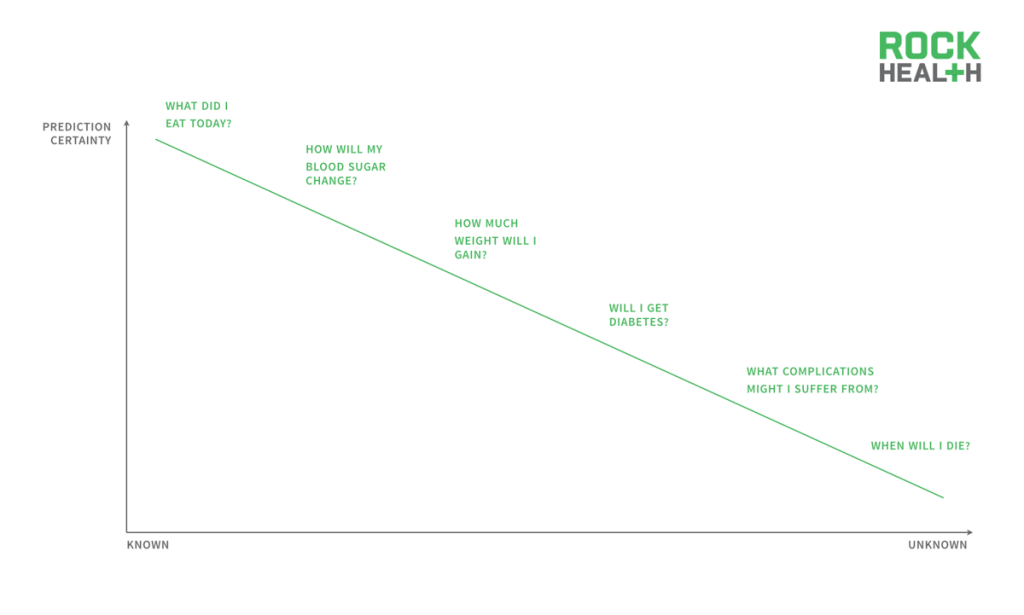
Prediction certainty changes with the type of question asked. For instance, asking a historical question such as “what did I eat today” is one that can be answered with a high certainty since the answer is “known.” However, as we start asking questions for events that have yet to happen, the prediction certainty decreases. Asking questions such as “how much weight will I gain” or “will I get diabetes” becomes harder to predict the answers with a high level of confidence.
In fact, “predictive analytics” underlies most of traditional medicine and health care, whether technology-enabled or not.
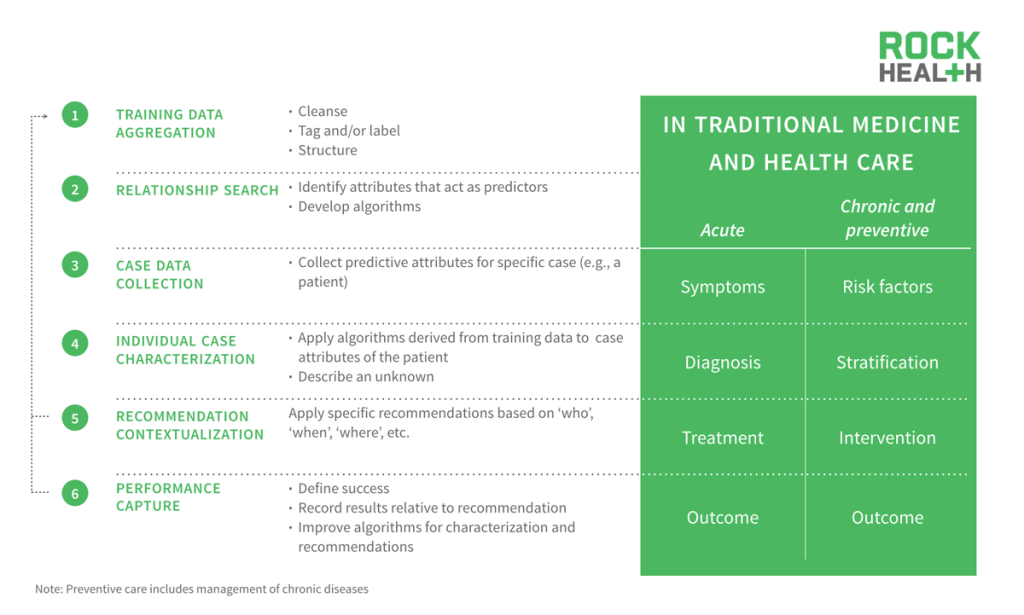
Most of traditional medicine and health care operate under “predictive analytics” today, driven by physicians’ minds versus software tools. The goal in bringing predictive analytics to medicine is to widen the training data set beyond an individual’s experiences so that individual patients can be better treated.
The overabundance of data and widespread availability of tools has catalyzed predictive analytics in health care.
Big data and algorithm production has reignited interest and excitement around predictive analytics. There has been an explosion of health care data—with new technologies to sequence our DNA, collect continuous monitoring data and patient-reported social media data, the amount of healthcare data is expected to grow to 25,000 petabytes in 2020. Thankfully, over the past few years, there have also been a wave of new technologies, including many open-source ones, that have emerged can process and manage all this healthcare data.
Investors certainly believe in the promise, pouring $1.9B into companies that purport to use predictive analytics.
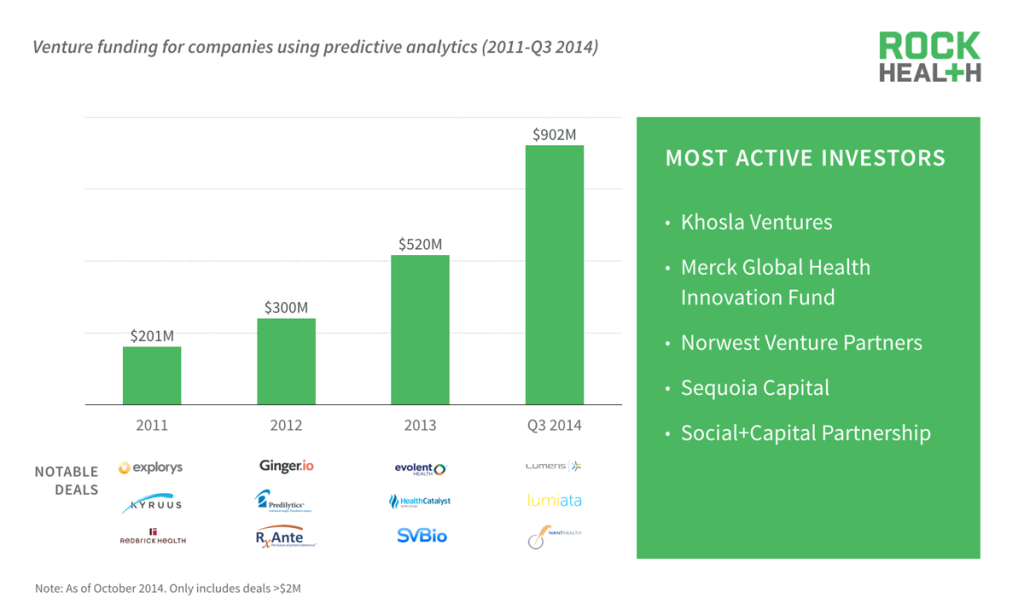
Source: Rock Health funding database
Interest in the promise that predictive analytics can help deliver better care while reducing costs has been increasing. Since 2011, over $1.9B of capital has been raised to fund companies that claim to use predictive analytics. Already in the three quarters of 2014 alone, funding has increased by almost 4.5x since 2011.
Funded companies claiming to use predictive analytics are highly focused on providers, practically ignoring patients.
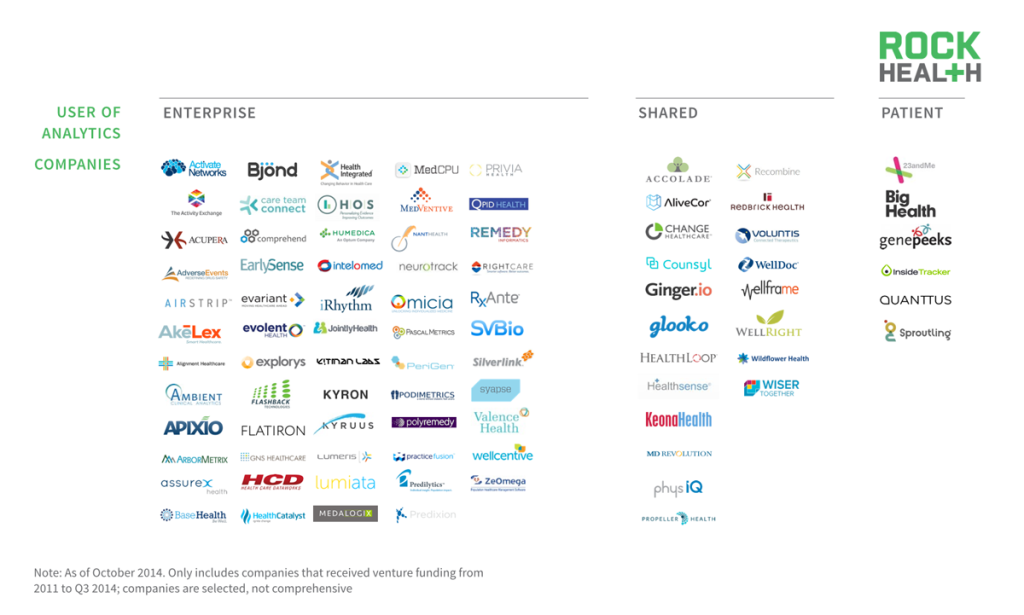
Source: Company websites
Of the companies that received funding between 2011 and 2014, majority of them focused on enterprise customers, specifically providers. These analytics and tools have designed to interface and impact the health care professional’s workflow, but with a growing list of companies that interact with both the provider and patient. However, there are few predictive analytics companies that are directed toward patients only.
New data streams, including those direct from patients, are beginning to be used by companies for predictive analytics.
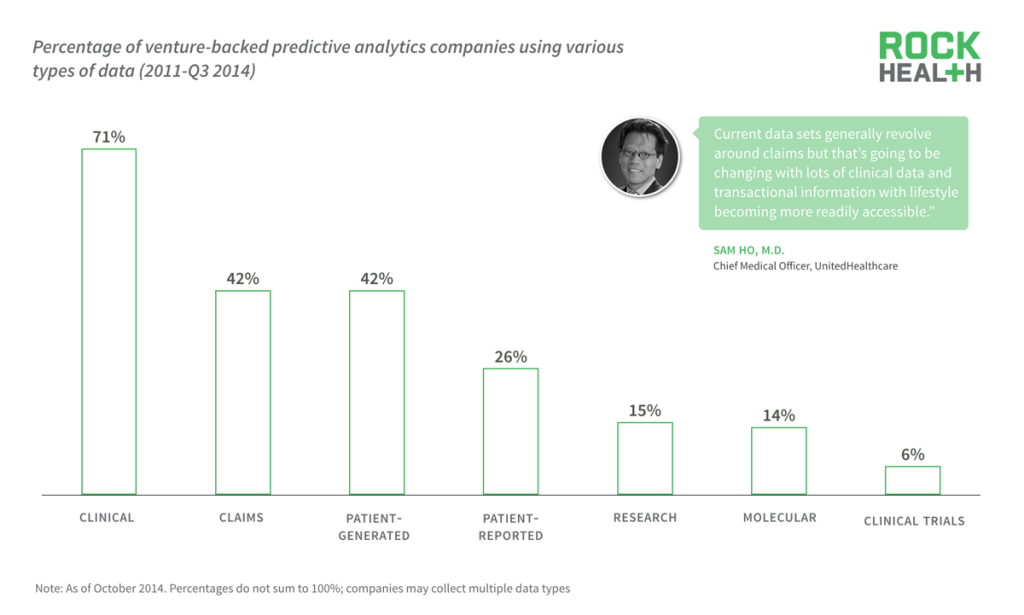
Source: Company websites
While the end-user is slowly starting to shift, new data streams, including patient-generated (such as continuous monitoring data from patches and wearables) and patient-reported (such as meal logging, mood logging, and social media), are increasingly being used for analytics.
Based on the stated or advertised data sources on each company website, an overwhelming majority, 71% of companies purport to use clinical data whereas only 42% of companies disclose using claims data. This is likely due to the fact that claims data has already been mined by companies for decades and emerging companies are trying to leverage new data sources to differentiate themselves.
Direction
Familiar methods of predictive analytics with a long history in other technology services are also appearing in health care.
Three categories of predictive analytics have been used and applied in other technology services, which are beginning to appear in healthcare. Here we show how Netflix has used all three themes of predictive analytics to enhance our tv/entertainment watching experience. But the consequences of a bad movie recommendation are trivial compared to the potential complications in healthcare.
Symptom calculators are the “recommendation engines” of health care, helping millions of consumers diagnose themselves.
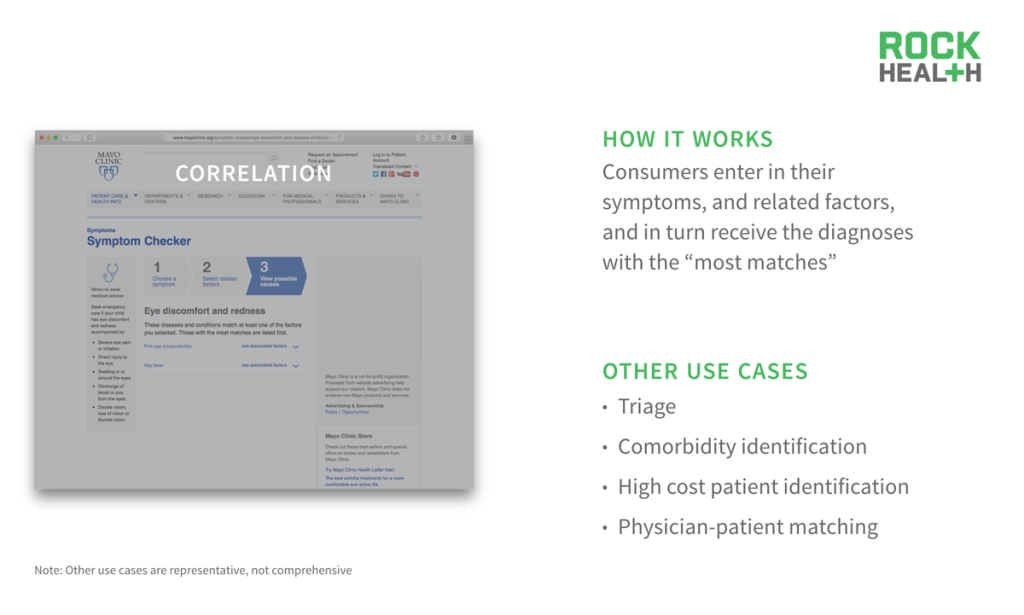
Source: Mayo Clinic
If you have ever gone online, entered your symptoms and received a diagnosis, you’ve interacted with healthcare’s version of a recommendation engine. Based on the symptoms and related factors you entered, the algorithm is matching you with others who have had similar inputs (i.e., your symptoms) and what diagnoses was most common. This type of analytics can also be used to help triage patients in high acuity situations, identify hidden co-morbidities, stratify and identify potential high cost patients, and help best match physicians with patients based on preferences.
Lacking appropriate context, clinical indicators—including vital signs—can generate false positives or negatives in alert systems.
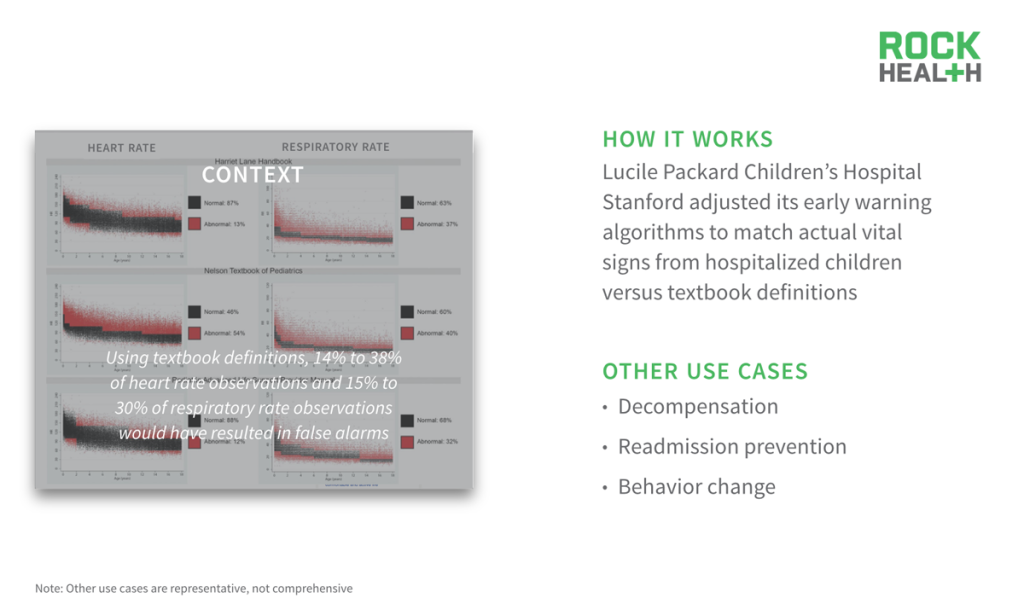
Source: Pediatrics (2013) and Health Affairs (2014).
The importance of having context around an individual’s historical data is so crucial, especially in healthcare where we are still learning what “healthy” or “normal” is. Textbook guidelines often provide ranges that are integrated into clinical alert systems without any context for each patient. For instance, data collected from the Cincinnati Children’s Hospital Medical Center and Children’s Hospital of Philadelphia showed that 14 to 38% of heart rate observations and 15% to 30% of respiratory rate observations would have resulted in false alerts based on textbook definitions. Lucile Packard Children’s Hospital Stanford has implemented these findings around their early warning algorithm systems.
It is crucial for these predictive models to be able to adapt their warnings to each individual. Other use cases where context will matter a lot in predictive analytics include decompensation, preventing readmissions, and behavior change. There is no silver bullet for behavior change because there is no universal context for patients.
Genetic screening companies similarly know the inherent risks before a child is conceived, allowing decisive action.
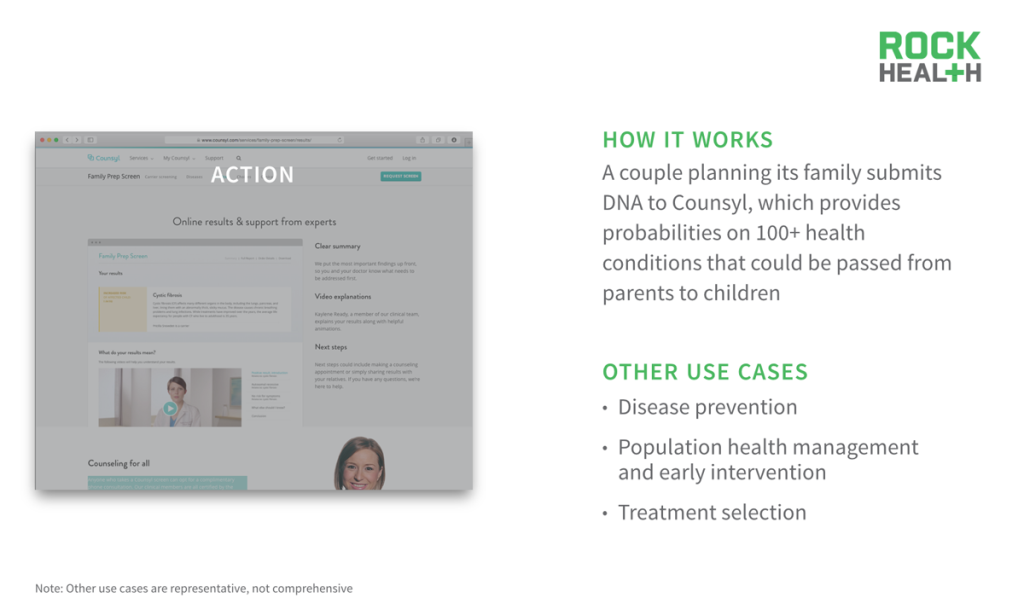
Source: Counsyl
As we think about how to best use analytics to result in taking action, genetic screening is a case example that allows parents to plan ahead. For example, a couple planning to have a family can send their DNA samples to Counsyl to understand the probabilistic risk of their child inheriting a certain disease. Use cases expand beyond just family planning. With large, smart data sets, you can run analytics to take action before you even “know” you need to such as disease prevention, population health management, early intervention, and even treatment selection.
Building models that break the curve of uncertainty will lead to personalized care, but it is not without significant challenges.
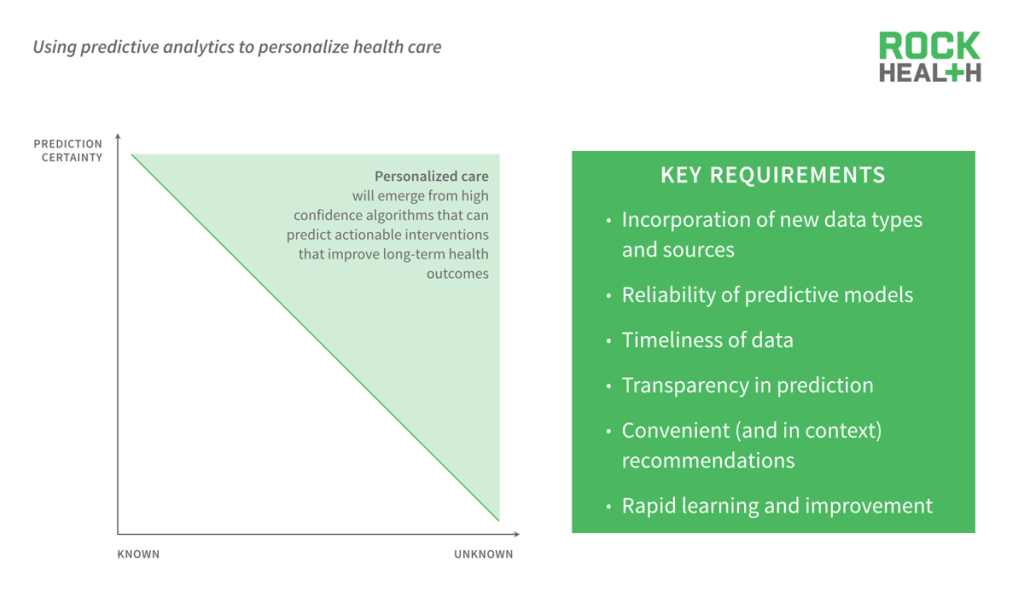
Predictive analytics can be used to improve the certainty of a prediction. Personalized care can emerge from high confidence algorithms that can predict actionable interventions that improve long-term health outcomes. However, building models that are able to reduce uncertainty and personalize care is not an easy task. It requires many factors such as the ability to incorporate new data types and sources, to have reliable, consistent predictive models, timely data, transparency around the prediction, convenience and contextual recommendations, and a closed feedback loop that allows the model to rapidly learn.
Challenges
The keystone of any successful predictive analytics model is the ability to improve the prediction based on a feedback loop.
Within seconds, Google knows whether its search engine prediction is correct. But in health care, the feedback loop—which is often measured in terms of impact on biometric or cost outcomes—can take years.
Startup companies are attacking the key challenges in predictive analytics, advancing the space and creating differentiation.
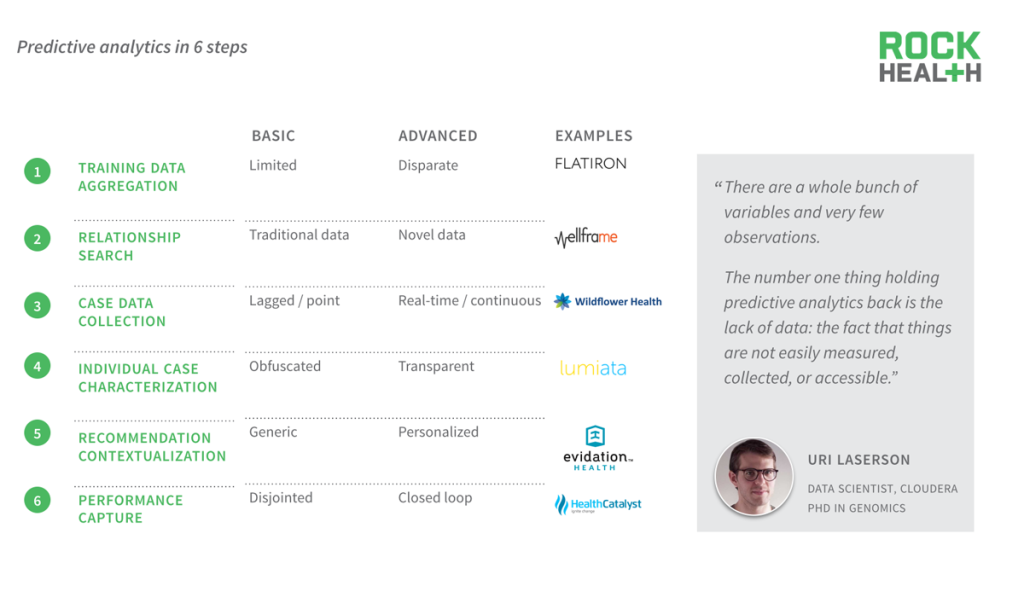
Emerging digital health companies serve as useful case studies for how each step in the predictive analytics process is advancing to be deeper, faster, and more personalized.
Six companies were reviewed to identify the challenges of advancing each step in the predictive analytics process.
Considerations
Healthcare providers don’t just want predictive analytics to output graphs and statistics. They need something that’s actionable.
You have to distill it down to what matters and is actionable. Because there’s a hundred thousand things that come into play in health care, predictive analytics has to tell us what matters and how we can act on it.
Personalizing care through predictive analytics represents a significant opportunity to reduce costs in the healthcare system.
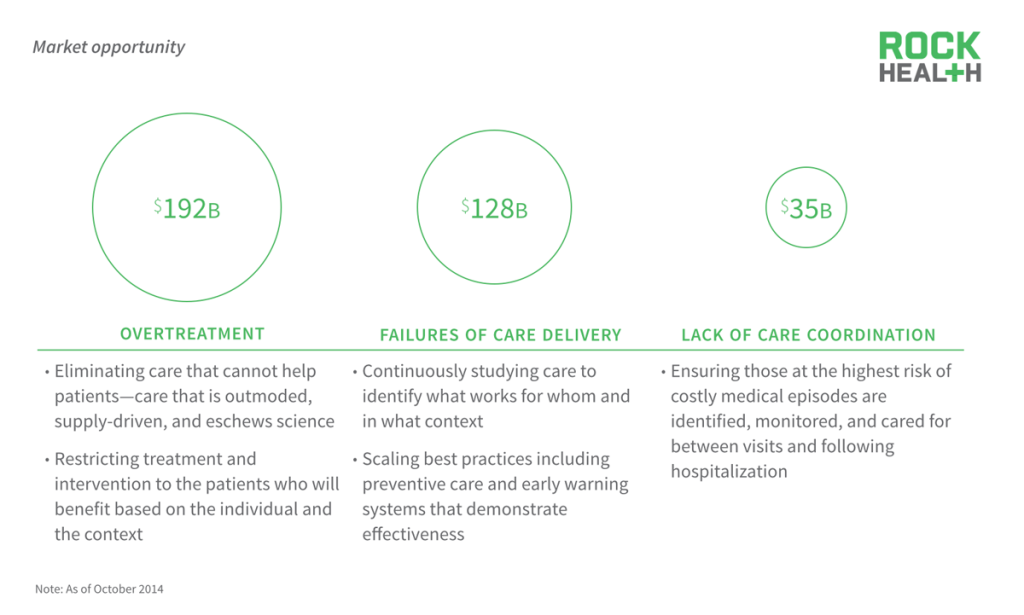
Source: Journal of the American Medical Association (2012)</span
There’s a massive opportunity for predictive analytics to improve care and dramatically reduce waste in the healthcare system, addressing systematic issues in over-treatment, care delivery, and care coordination.
It will largely fall onto the healthcare industry to recognize the value of predictive analytics and implement critical use cases.
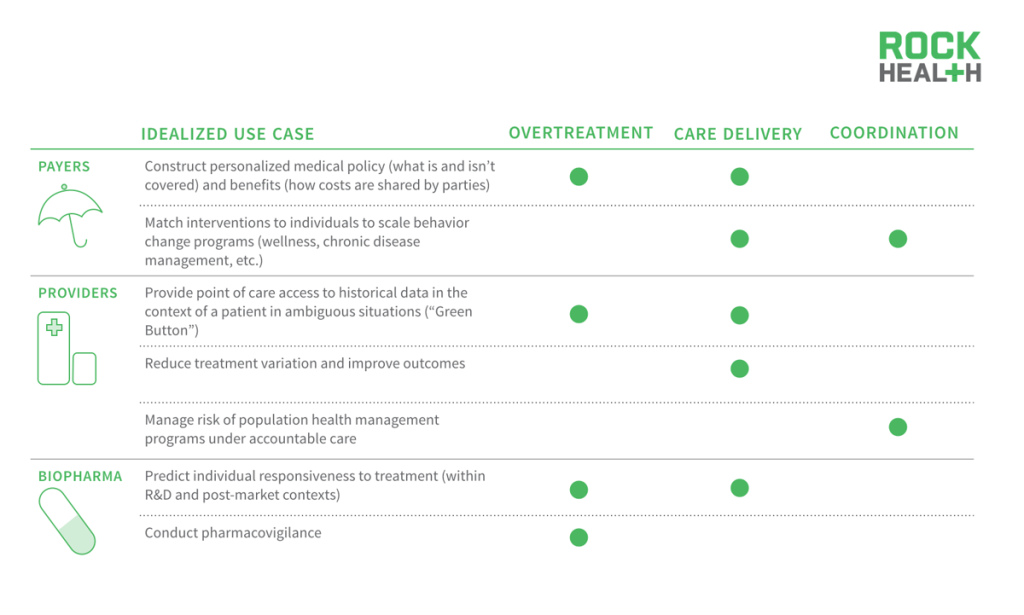
The entire healthcare industry could benefit from the usage and adoption of predictive analytics. For example, payers could use it to construct personalized medical policy. Today there are global policies for what is and isn’t covered, which physicians are in and out of network, but perhaps it should be based on what treatments make the most sense for each individual patient.
The industry might be waiting to implement predictive analytics as the FDA decides how best to regulate clinical decision support.
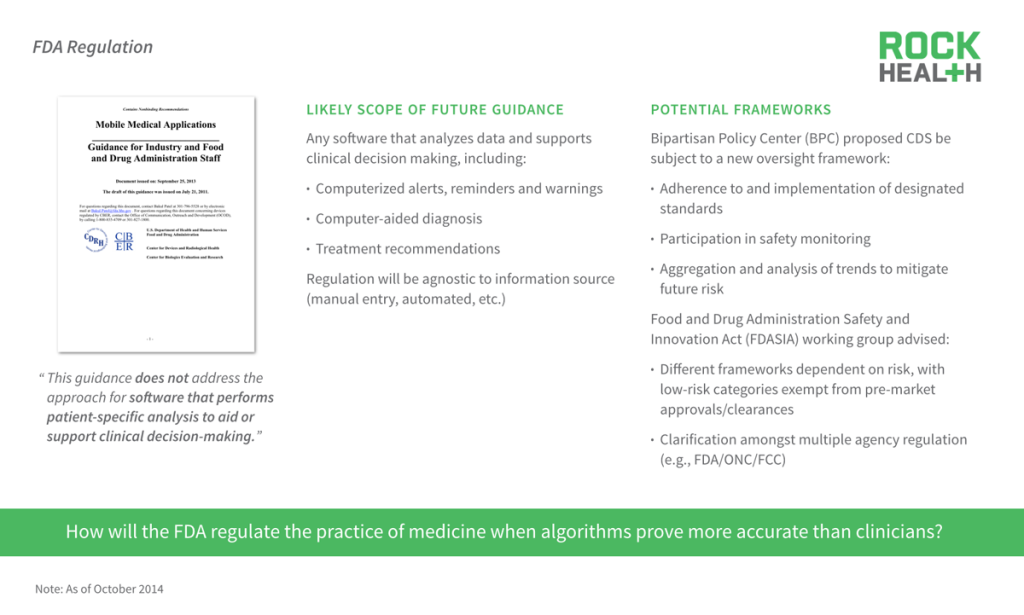
Source: FDA.gov and Journal of Law and the Biosciences (2014)
Future guidance will need to cover critical issues within clinical decision support, including alerting systems, computer-aided diagnosis (CAD), and direct treatment recommendations.
A number of potential frameworks have emerged, including those from the Bipartisan Policy Center (BPC) and the Food and Drug Administration Safety and Innovation Act (FDASIA) working group, all of which will need to be reconciled into final guidance that incorporates a potential new reality: algorithms that are more accurate than physicians.
Beyond regulation, the biggest risk to predictive analytics being used in health care is adoption as power dynamics shift.
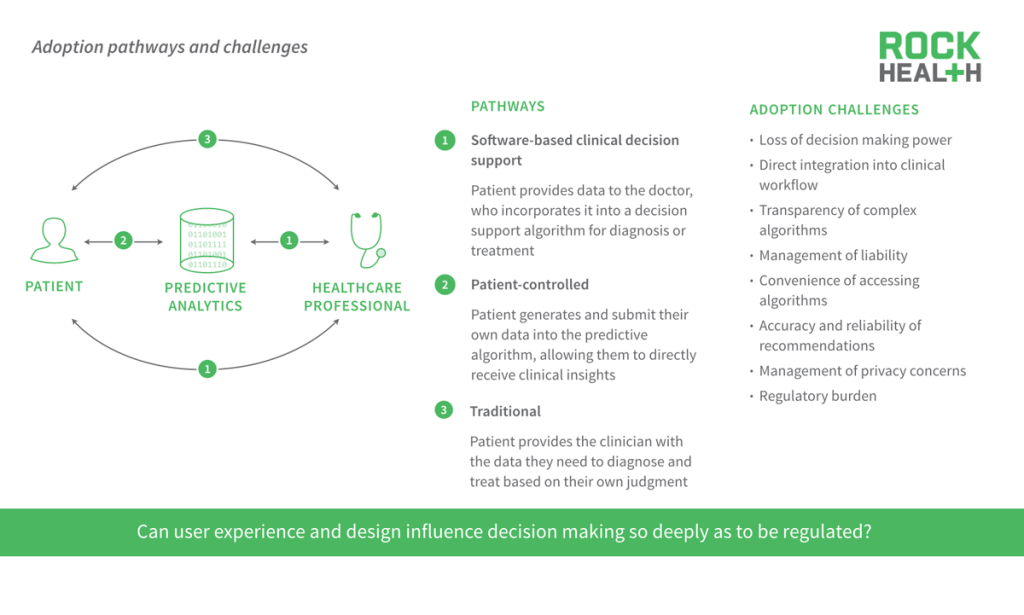
Leveraging predictive analytics can change the power dynamics between patients and physicians. If physicians don’t readily adopt predictive analytics for fear of losing decision-making power or liability concerns, then patients will go straight to the algorithms to find their own answers. But consumers need to trust the accuracy and reliability of these recommendations. We’re excited and waiting to see those WebMD 2.0 companies that use real data from patients to produce more accurate recommendations.
One of the largest challenges is adoption. Medicine is shifting to a probabilistic model that will give weighted treatment recommendations. How do physicians decide which recommendation to pick? Design is about manipulation, so how recommendations are presented will significantly influence the decision-making process. FDA will face concerns around regulating user experience to ensure that design layouts are not abused.
Overcoming these technical, regulatory, and adoption hurdles will not be an easy task. But until then, we’re optimistic that predictive analytics will continue to make advances and deliver personalized care.
We are underestimating the potential impact of predictive analytics in process tools to help physicians make better decisions.
Every week, at the airport, I get on an airplane, and I don’t worry about flying at all. There are so many tools deployed to assist the pilot. I was talking with a pilot about the new 787–and the pilot said he basically monitors the plane. We’re going to see more of that in health care.
Physicians will be monitoring algorithms.
Acknowledgements
We are indebted to our industry partners who not only support our work every day but provided invaluable feedback on an early draft of this report.
A number of industry, startup and venture folks also offered their expertise. Special thanks to Karina Babock, Benjamin Berk, Archit Bhise, Joe Boyce, Matt Butner, Chris Coloian, David Crockett, Ash Damle, Asif Dhar, Bill Evans, Kevin Fickenscher, Luca Foschini, Ryan Goldman, Josh Gray, Sam Ho, Lucian Iancovici, Anil Jain, Donald Jones, Allen Kramer, Uri Laserson, Christine Lemke, Dave Levin, Dan Martich, Phil Okala, Trishan Panch, Vinnie Ramesh, Leah Sparks, David Tamburri, Euan Thomson, Abhimanyu Verma, Nate Weiner, and Jack Young for their time and insights.
Finally, we are fortunate to work with the most encouraging and passionate team in digital health. We are certain that no one would even be reading this report if not for the tireless marketing efforts of Halle Tecco and Mollie McDowell.