The emerging influence of digital biomarkers on healthcare
For full access to the report and all of our research, become a Rock Health partner—email partnerships@rockhealth.com.
Healthcare is undergoing a technological transformation, and it is imperative for the industry to leverage new technologies to generate, collect, and track novel data. With the wealth of new data, the onus is on the system to turn it into relevant information that helps researchers, clinicians, entrepreneurs, and consumers better understand states of both disease and health. Digital biomarkers are an opportunity to translate new data sources into informative, actionable insights. In this report, we provide an overview of and glimpse into the emerging space of digital biomarkers.
The path to adoption is not an easy one. Digital biomarkers will, at a minimum, face the same regulatory challenges as traditional biomarkers. But not all digital biomarkers are created equally. There is great potential to apply digital biomarkers to medical domains that are not well understood, such as psychiatry and neurology, especially if digital biomarkers are combined into phenotypic signatures. Establishing insightful relationships is only the first step before the industry can truly capitalize on the value of digital biomarkers. Challenges around evidence generation, infrastructure, incentives, and workflow remain.
The question remains to be answered—will digital biomarkers be better tools to help understand health and disease?
Background
Digital biomarkers are consumer-generated physiological and behavioral measures collected through connected digital tools.
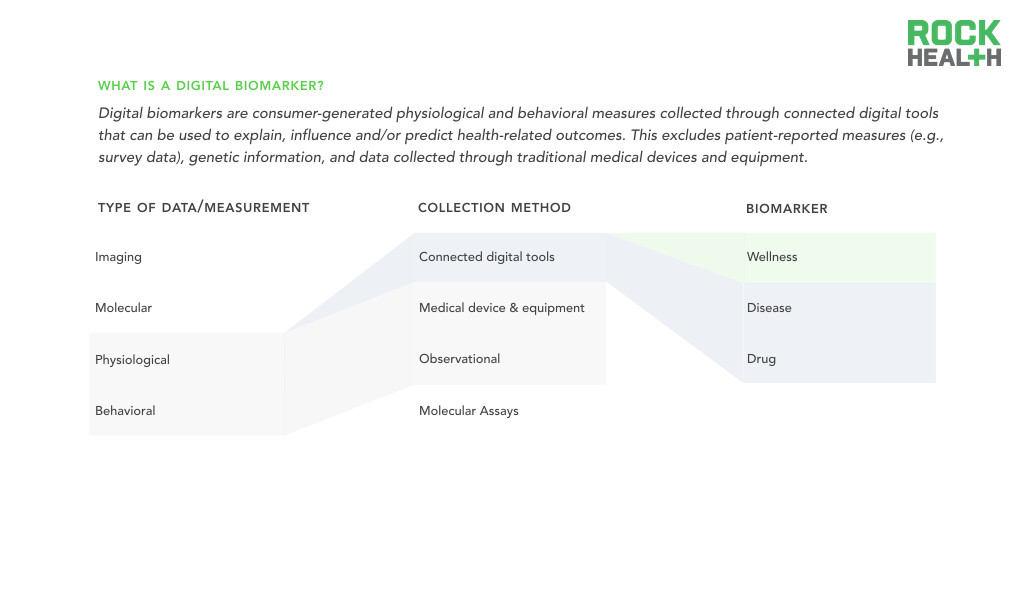
Note: Scope and definition of digital biomarkers is for the purposes of this report
According to the NIH, biomarkers are objectively measured and evaluated indicators of normal biological processes, pathogenic processes, or pharmacologic responses to a therapeutic intervention. But as the world has gone digital, medicine now has access to a new type of biomarker. Digital biomarkers are consumer-generated physiological and behavioral measures collected through connected digital tools that can be used to explain, influence and/or predict health-related outcomes. Health-related outcomes can vary from explaining disease to predicting drug response to influencing fitness behaviors. In our definition of digital biomarkers, we exclude patient-reported measures (e.g., survey data), genetic information, and data collected through traditional medical devices and equipment. These data types, though still a key component of research and clinical care that may be stored digitally, are not digitally measured or truly dependent on software.
Digital biomarkers are classified by novelty in measurement and insight.
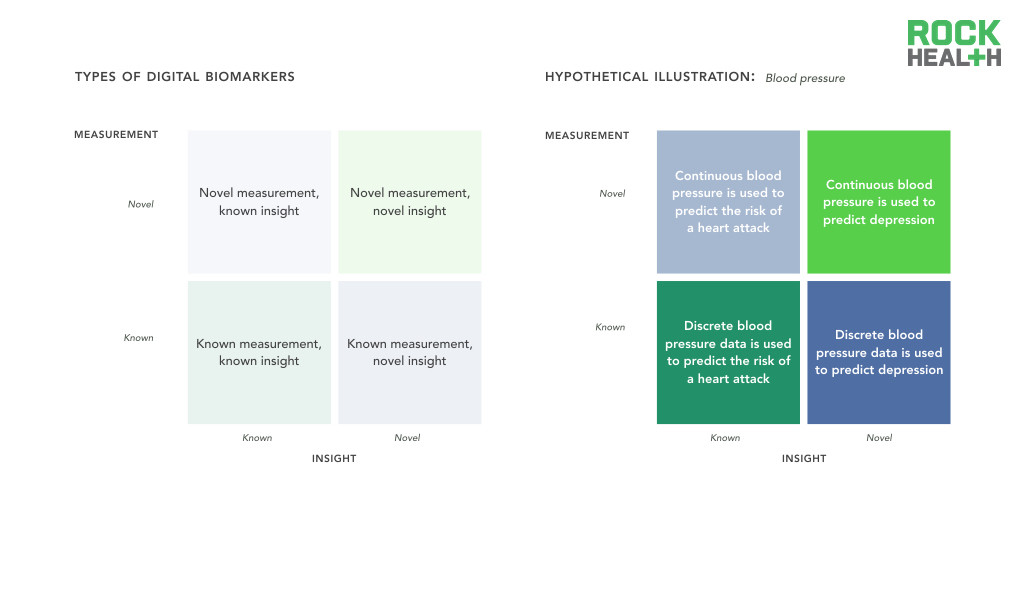
Note: Assumes all measurements are captured / acquired via connected digital tools
Digital biomarkers are a priori collected by digital means, therefore we believe the most effective classification of these measures focuses on what is being measured and the clinical insight derived from that metric. Measurements can be known, such as discrete measurement of blood pressure, or novel, such as continuous measurement of blood pressure. A known insight is one that has previously been validated and is well understood. For example, blood pressure (a known measurement) can be used as an indicator of a known insight such as cardiovascular risk. Alternatively, the known measurement can be used to discover a novel insight linking blood pressure to major depression. These digital biomarker categories will likely inform the level of evidence required for validation and regulatory approval.
Consumer-facing digital tools, both mobile health apps and sensors, that can generate health data are on the rise.
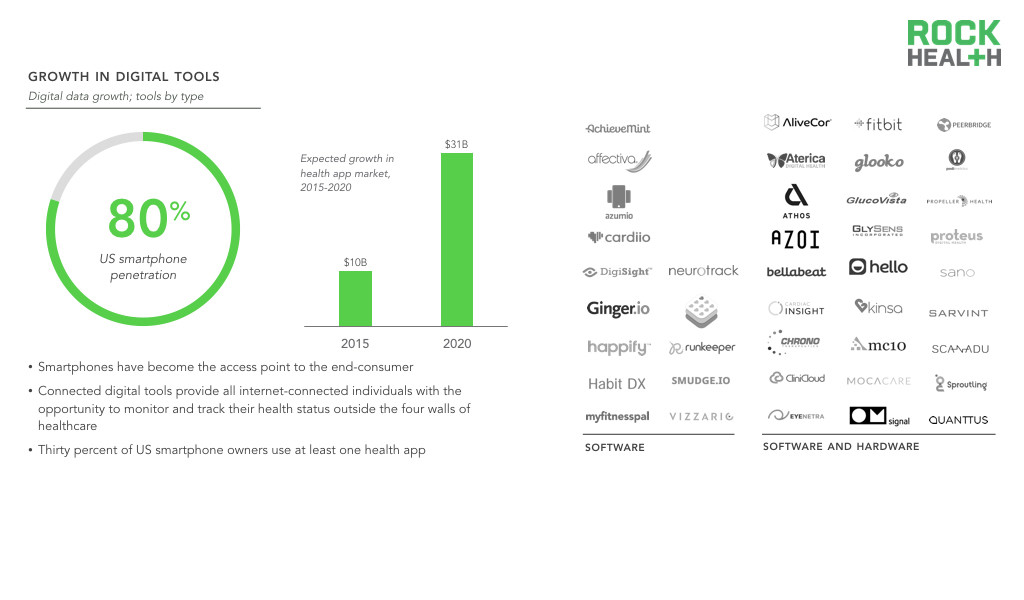
Source: Nielsen, research2guidance; Digital Health Consumer Adoption; Company websites
Note: List of digital tools were selected, not comprehensive
The growth in healthcare data can be attributed to broader access to and adoption of digital tools as well as the ability for these tools to allow physicians to continuously monitor individuals. Consumer-facing digital tools expand the population that can generate health data. Individuals who were not “patients” or formally enrolled in clinical research were previously excluded from the data collection processes, but digital tools provide all internet-connected individuals with the opportunity to monitor and track their health status outside the four walls of healthcare. For instance, while traditional blood pressure cuff monitors provide static, infrequent measurements, wrist-worn devices can continuously record vitals over time with minimal effort from any end-user.
Smartphones have become the access point to the end-consumer. Thirty percent of US smartphone owners use at least one health app, many of which allow individuals to track various health measures (e.g., blood pressure, heart rate, physical activity, sleep). Researchers are also leveraging smartphones to reach a large population—more than 50 researchers have already contributed to Apple’s open source ResearchKit platform, and over 100,000 individuals around the world have chosen to contribute their data to advance research.
Many digital health technologies enable more convenient, cost-effective means to collect discrete health measures, such as blood pressure and glucose, that clinicians can draw upon in decision-making processes. For example, AliveCor is an iPhone case that acts as a tool for consumers to take an electrocardiogram (ECG) at home or on-the-go. The ECG reading can be interpreted by a physician (or algorithm) to diagnose cardiac arrhythmias.
Additionally, the digital footprint that consumers leave when they engage with the internet, through web browsing or social media activity, provides novel data that can be leveraged for healthcare purposes. Consumers on sites such as Facebook and Twitter are frequently willing to share their social media data, allowing researchers to draw correlations between this data and health outcomes. As other types of consumer data continue to be collected digitally (e.g., shopping behavior tracked through mobile apps), the industry has the opportunity to leverage the resulting data to create more holistic pictures of an individual and tailor care plans accordingly.
Data becomes a digital biomarker when a relationship is drawn to a health-related outcome.
When paired with analytical tools, these large volumes of data can be leveraged to track trends and patterns for both individuals and populations. Demonstrated correlations between data (e.g., physical activity, heart rate) and clinical outcomes (e.g., hospitalization for cardiovascular disease) can be used to alert consumers to take precautionary steps or present themselves to a clinical care setting for high-acuity conditions. Health systems can apply these insights to deliver the optimal treatment at the best time to each patient, including better identifying and managing high-risk, high-cost patients. For instance, a biosensor recording and analyzing intestinal vital signs is used to predict whether abdominal surgery patients will have postoperative complications.
Digital biomarker creation requires direct access to and the ability to integrate longitudinal medical, behavioral, and contextual data. After that, retrospective analysis works well as a first step in developing and testing hypotheses, but prospective clinical studies are critical to validating the hypotheses and developing robust evidence of the digital biomarker’s value.
The Value of Digital Biomarkers
Longitudinal data collection at both the n of 1 and population level allows for high resolution digital biomarkers.
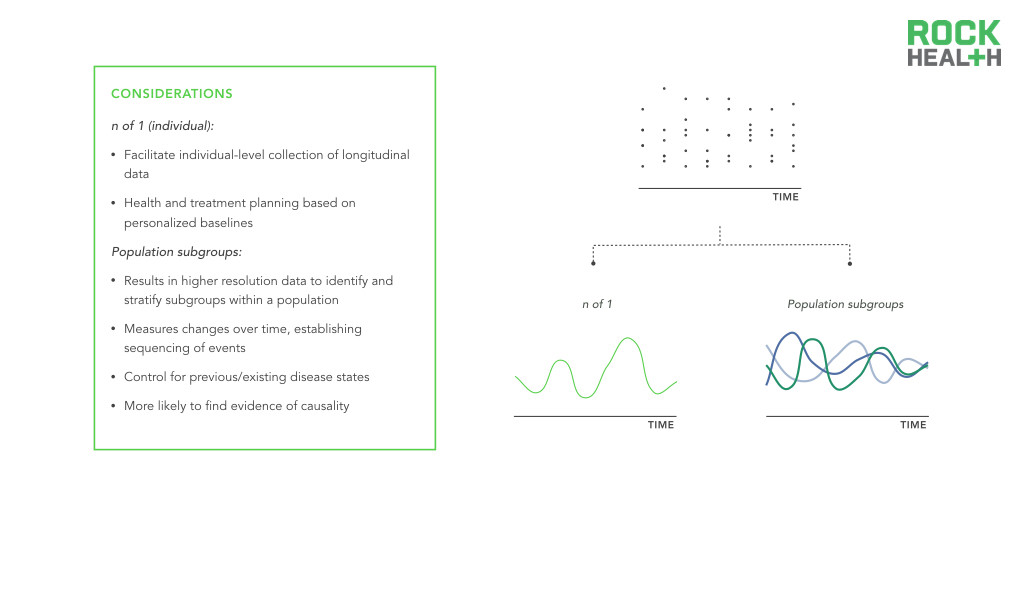
Longitudinal data offers invaluable information to better understand, detect, and manage diseases. Seminal investigations like the Framingham Heart Study, the Nurses’ Health Study, and the Women’s Health Initiative typify the power of longitudinal data. However, these studies are difficult to implement and fund. Moreover, they do not fully capture demographic heterogeneity. Digital biomarkers offer a cost-effective opportunity to extend the collection of population level health data over time and introduce longitudinal data for individual consumers. Through passive and continuous monitoring, it becomes possible to collect real-world data over extended periods of time. This data can be used to guide an individual’s care or it can be combined with data from other individuals to enable more precise population health management.
Digital biomarkers represent an opportunity to capture clinically meaningful, objective data in a cost-effective manner.
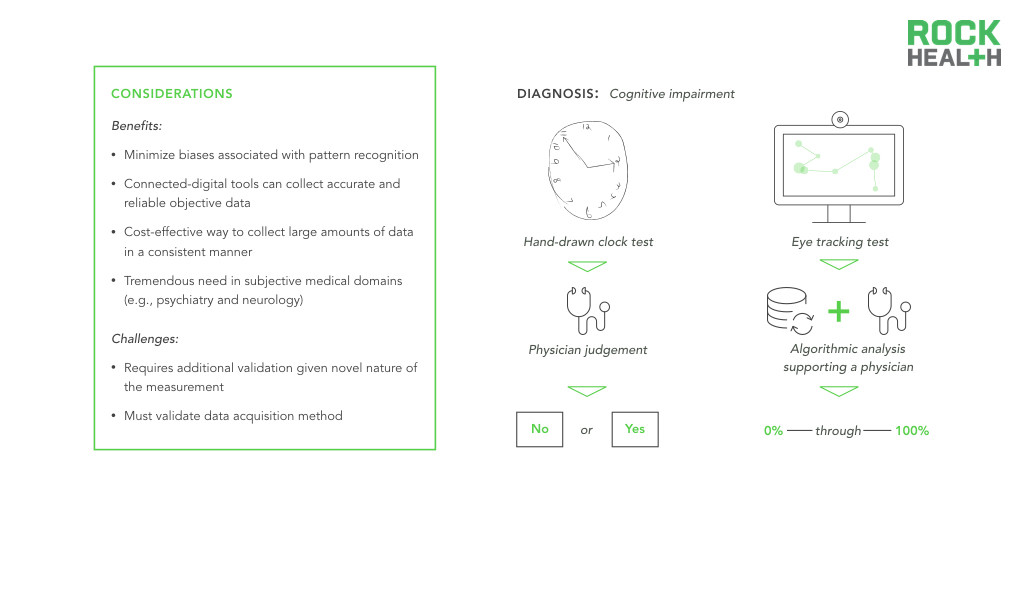
Digital biomarkers have the greatest opportunity to supplement existing biomarkers in medical domains that still heavily rely on subjective and observational assessments to diagnose or monitor disease. Take for example psychiatric and neurological conditions. While some neurological conditions have definite physical manifestations, disorders of the brain inhibit clinicians from utilizing solely empiric evaluations. To understand an individual’s mood or to measure the disordered thought process of a patient with schizophrenia, clinicians use behavioral and psychosocial assessments, which vary in sensitivity and specificity. Observational or interview-based behavioral assessments present ample opportunity for excess subjectivity to be introduced into care. Neurology and psychiatry represent fields in which objective gold standard metrics are difficult to establish and even harder to maintain. Digital biomarkers that passively measure digital and online behavior represent an opportunity to develop more objective measures of brain disorders.
A combination of digital biomarkers enables the discovery of phenotypic signatures to better explain variance in human health and disease.
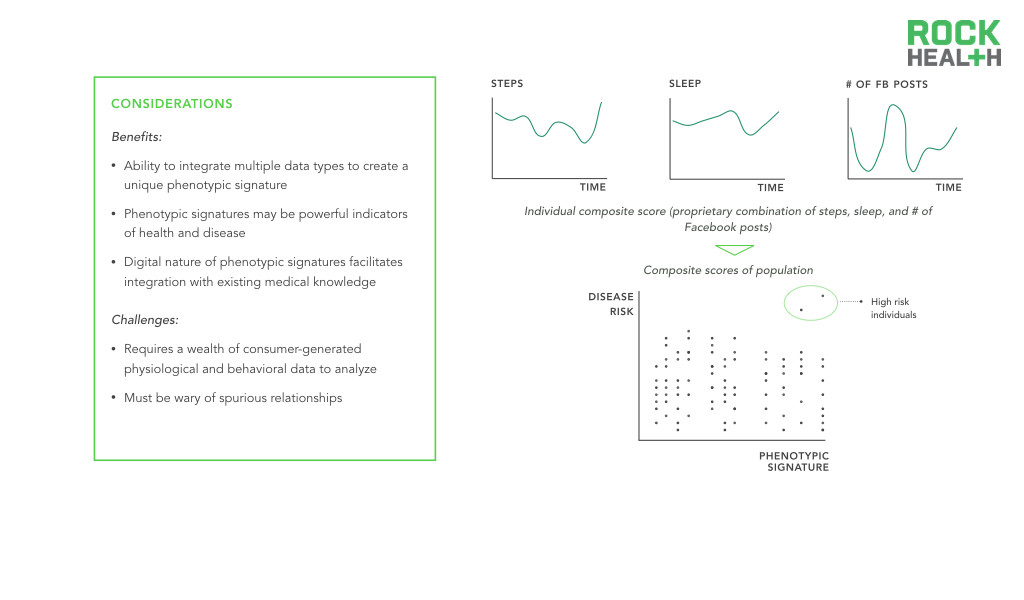
The most promising consequence of digital biomarkers is the ability to create digital biomarker panels. In medicine, single discrete measurements have limited clinical significance—10 year cardiovascular risk is best predicted by a set of measurements including age, gender, cholesterol levels, smoking and medication status, and blood pressure. Integrated panels of digital, and traditional, biomarkers offer an opportunity to better explain variance in human health and disease. A parallel can be seen in the advent of gene expression signatures that serve diagnostic, prognostic, and predictive roles—researchers are able to identify a set of genes that act together in disease states, producing a genetic biomarker. Similarly, phenotypic signatures can be developed by identifying a set of biomarkers that additively enable precise monitoring of disease states.
A further integration step will occur when digital phenotype signatures are combined with other types of data. Data integration is nascent. Take for example a recent study which used EMR data mining to discover clinical subtypes of Type II diabetes and integrated genetic association analysis to identify genetic markers of each subtype. Combining such efforts with validated digital phenotype signatures promises to directly enhance diagnostic, prognostic, and predictive capabilities.
Digital biomarkers turn the evidence generation and validation pathway into a closed loop.
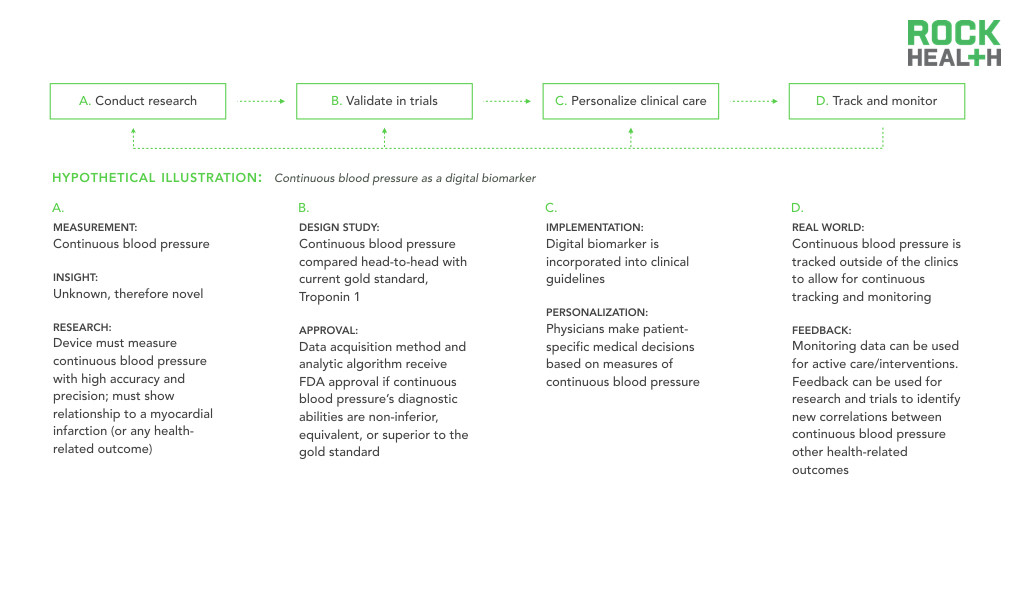
Before vast amounts of data combined with sophisticated algorithms can deliver improved outcomes, validation through testable hypotheses and replicable results is necessary. The promise of digital biomarkers is firmly tied to the ability of digital health entrepreneurs, researchers, and other digital biomarker developers to produce compelling evidence-backed claims.
For academia and research institutions to analyze data and identify relationships for new digital biomarkers, exploratory hypothesis testing must occur. Once a potential relationship is drawn, the majority of industry experts maintain that digital biomarkers will follow a similar approval path to traditional biomarkers. Thus, the adoption of digital biomarkers in clinical practice will be contingent upon rigorous validation and approval. If, and when, digital biomarkers are integrated and used in clinical practice, this will yield a new set of evidence to verify and validate conclusions drawn using data from controlled settings.
Historically, real-world outcomes have been the most difficult to track and feed back into the healthcare system, which limits the medical community’s ability to constantly reevaluate clinical protocols. Without insight into how patients are responding to a drug or treatment in the real-world, healthcare professionals cannot adjust and improve tailored care plans. By leveraging digital biomarkers, providers can essentially run a “trial” for each individual patient, continuing to generate evidence for care protocols.
Digital biomarkers will extend and amplify the consumer’s role in his/her own health.
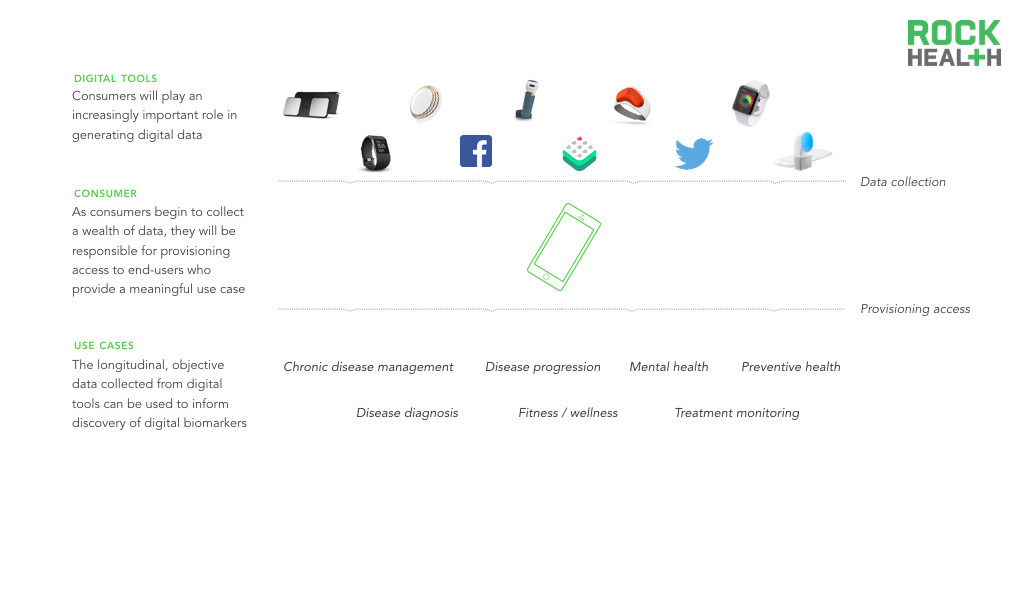
Source: CDC; Company websites
As consumers collect more types of health data (e.g., medical records, lab data) and become accustomed to controlling their own health data, each individual can begin to serve as a central data aggregator. Digital biomarkers are collected through consumer-facing tools, positioning consumers to be able to provision access to third-parties, including providers. Thus, digital biomarkers provide an incredible opportunity for fluid data transport with ownership of data shifting from the industry to the consumer. For instance, patients using MyHeartCounts can provide consent to share collected daily activity and cardiovascular health information with researchers at Stanford. As central data aggregators, consumers can then provision access to relevant stakeholders.
Moreover, with nearly half of all adults in the United States having at least one chronic disease, behavior becomes one of the most important determinants of health. Consumers must learn to actively manage their health. Because digital biomarkers can be the resulting “insight” of objective behavioral data, this represents an opportunity for physicians to impact behaviors to provide the best care plans and interventions.
The industry can access and better understand a holistic view of an individual’s health when leveraging digital biomarkers.
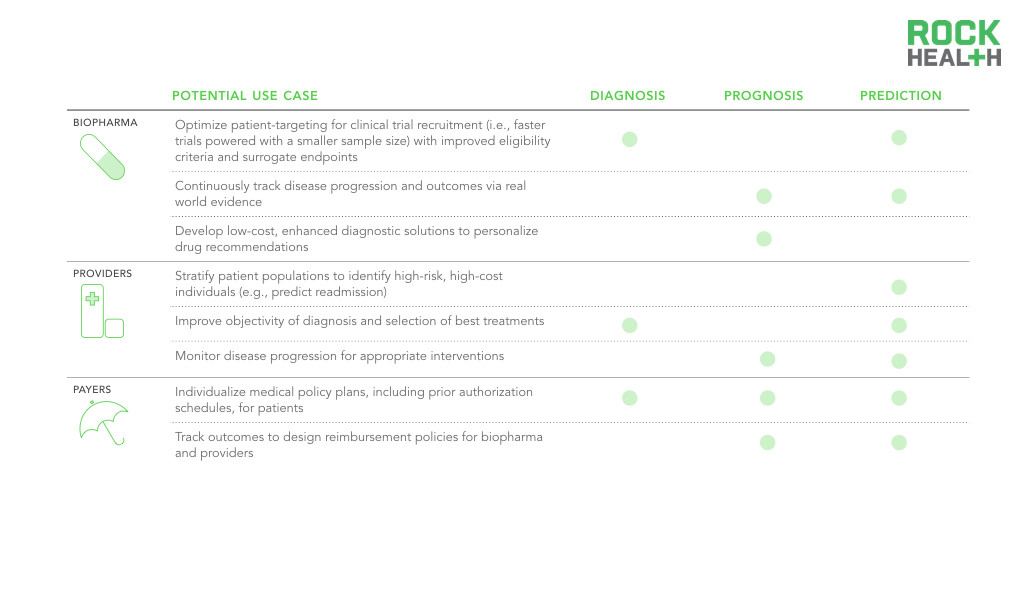
Note: Potential use cases are not comprehensive, reflect high priority use cases
Biopharma will need to leverage digital biomarkers to demonstrate increased real-world value and benefit as the shift towards pay-for-performance increases. This motivates biopharma to improve patient outcomes with enhanced stratification guidelines and improved surrogate endpoints using digital biomarkers. Having access to each individual patient through the smartphone presents an opportunity for unprecedented engagement and ability to monitor disease progression with novel digital biomarkers. With a better understanding of disease progression, biopharma and providers can work together to intervene sooner at the onset of symptoms.
Clinical protocols that drive treatment decisions are used across general disease populations, but usage of digital biomarkers can help shift to a more personalized approach. Providers can apply digital biomarker insights to deliver the optimal treatment at the best time to each patient, including better identifying and managing high-risk, high-cost patients. For instance, digital biomarkers could be used to predict readmissions as well as to identify the best interventions for a specific patient. Moreover, because digital biomarkers are generated outside the four walls of the healthcare system, they provide a window into a patient’s day-to-day status between physician visits. Digital biomarkers can be used as a collection of real-world information, analyzed in conjunction with clinical and molecular data, and returned to the healthcare professional in real-time, enabling precise, patient-specific medical decision-making.
Additionally, payers can use digital biomarkers to further stratify patients and create individual care plans, including prior authorization schedules. Individual or groups of digital biomarkers that are shown to be superior, rather than equivalent, to existing measures will more likely receive reimbursement and drive provider adoption. Healthcare professionals must be properly incentivized (e.g., through value-based payments and billable codes) to invest in the proper infrastructure and workflow integration. Reimbursement approval will play an instrumental role in the adoption of this new collected data.
Challenges
Collecting data is the ‘easiest’ part of the process. Linking the data to some relevant phenotype is the hardest part—what clinical outcome can we predict and what can we associate with it?
Before data becomes an insightful digital biomarker, many challenges still exist around identifying and processing relevant data.
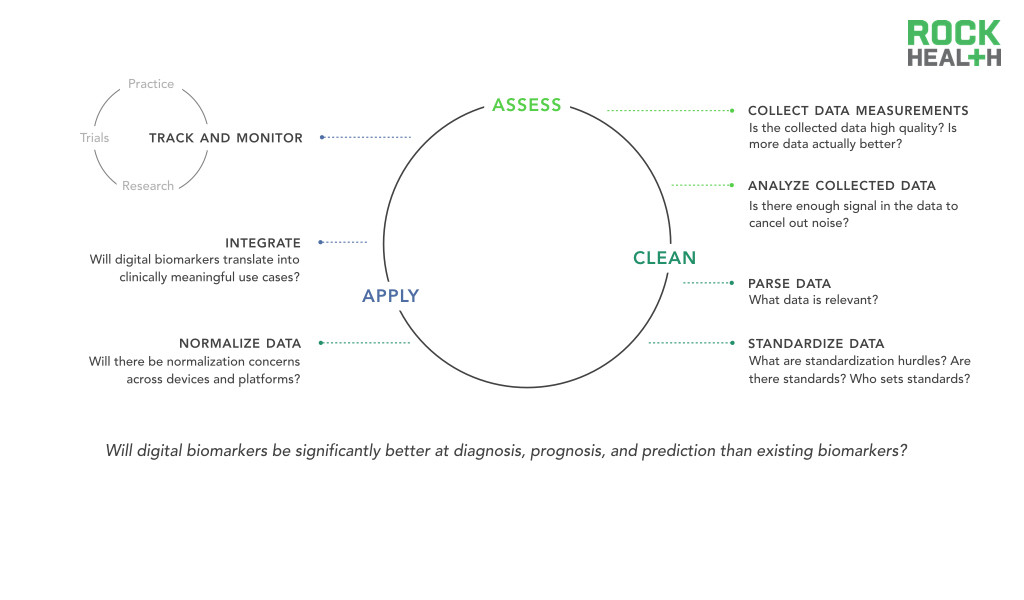
While the data collection of continuous and novel measures is one of the most exciting promises of digital biomarkers, it also faces one of big data’s quintessential questions—is more data actually better?
As discussed, there are specific clinical domains, such as sleep disorders, in which continuously-collected digital biomarkers already have clear promise in replacing traditional methods of data collection (polysomnography). However, the advantage of an uninterrupted data stream is not as straight-forward in other areas. For instance, it is unknown if around the clock tracking of oxygen saturation will have meaningful predictive and prognostic implications. Expert opinion reveals confidence that the analytic tools to effectively parse continuous data streams exist or can be developed with relative ease. What remains unclear is whether digital biomarker-generated data can be associated with health and disease. Continuous blood pressure monitoring may provide a new level of cardiovascular monitoring or it may be more indicative of mood and emotion. Currently, we have little evidence to prove either case. In healthcare, big data is only valuable when there is a strong body of clinical evidence.
Related challenges exist in data hygiene and standardization. If datasets are not properly cleaned and maintained in standardized forms, integration between different digital biomarkers and between digital biomarkers and existing healthcare infrastructure (e.g. EMR) will be significantly inhibited. The field of genomics and genetics has agreed to certain standards of data reporting and sharing that facilitate integrative analyses and the development of consensus gene signatures. Without similar initiatives, digital biomarkers face barriers to comprehensive integration.
Research must be done to validate digital biomarkers as accurate and reliable.
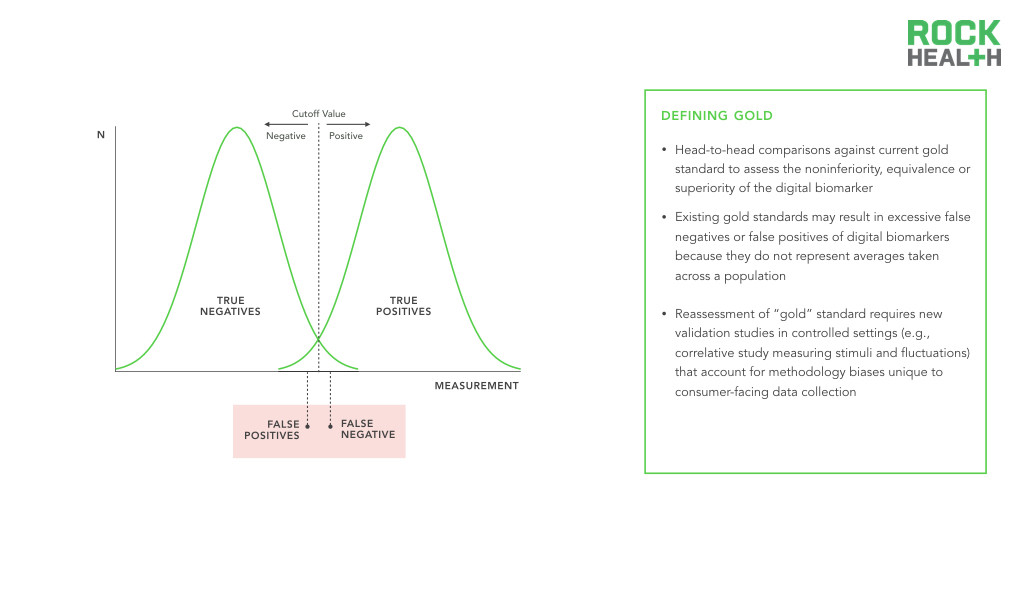
Before regulatory approval, the digital biomarker will need to be supported by evidence that demonstrates its specificity, sensitivity, and positive and negative predictive values. Furthermore, the digital biomarker will likely need to be evaluated head-to-head against the current gold standard to assess the noninferiority, equivalence or superiority of the digital biomarker.
However, the development of digital biomarkers which are able to collect individual-level evidence raises a challenge in validation—what if the gold standard is not really a “gold” standard? Traditional biomarkers, which will serve as the gold standard for comparison, have baselines and cut-off points established by averages across large, heterogeneous populations. Comparing these measures head-to-head with digital biomarkers may not be a fair assessment. Such an apples-to-oranges comparison opens the door to false negative results, where the digital biomarker produces a real result, but is wrongly judged to be false because of the inappropriate comparison with the traditional gold standard. Given that digital biomarkers offer personalized metrics and the creation of an individual baseline for physiological and behavioral processes, comparisons must avoid rejection of digital biomarkers simply because they do not represent averages taken across a population.
Traditional clinical trials regard double-blinded, randomized controlled trials as the gold standard. However, given the consumer-centric nature of digital biomarkers, this may create biases. For example, the act of tracking a metric inherently changes patient behavior and outcomes, which may skew the population set for digital biomarkers. Therefore, there is a need to consider alternative validation strategies for digital biomarkers. One option may be to design well-controlled correlative studies in which multiple small stimuli are introduced and the ability of a digital biomarker to detect these fluctuations is assessed. This would be similar to a dose-dependency study, where a stepwise pattern in a particular phenotypic measure can be established, much as treatment response to a series of drug doses can be used as evidence that the drug is producing the desired effect.
Like traditional biomarkers, digital biomarkers face a long regulatory pathway to approval.
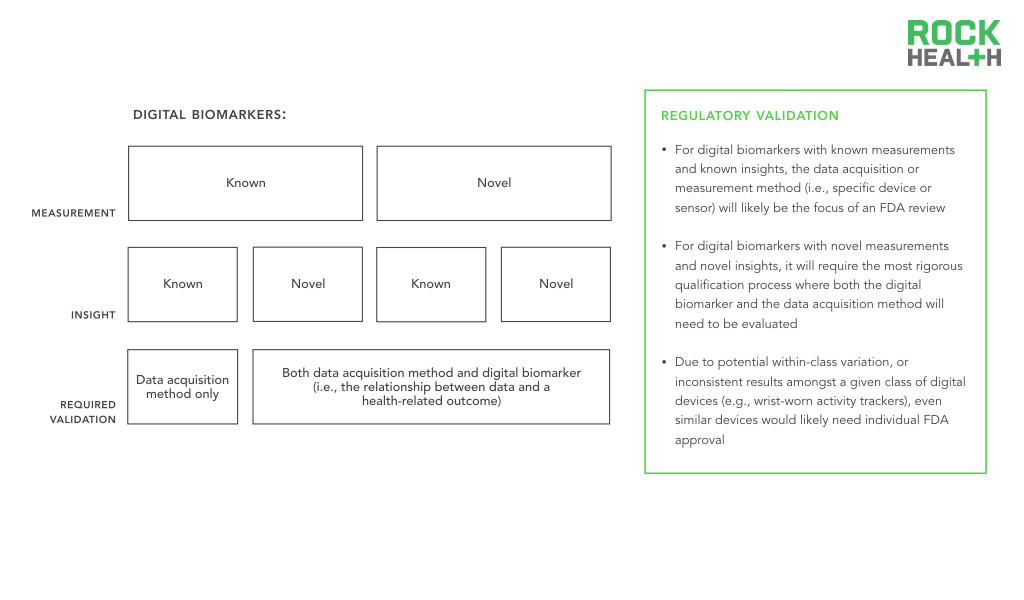
Regardless, the four types of digital biomarkers require some degree of validation. Best practice dictates that entrepreneurs and researchers should engage in conversations with the FDA earlier rather than later. For known-measurement / known-insight digital biomarkers, the measurement method (i.e., specific device or sensor) will likely be the focus of an FDA review, as the biomarker itself, collected traditionally, has been previously validated. Digital biomarkers with novel measurements and novel insights will require the most rigorous qualification process as both the biomarker and the measurement method will need to be evaluated. For example, to establish the superiority of continuous over discrete measurements in clinical trials and clinical care, the value of continuous data collection would need to first be established (e.g., better identifying individuals at risk for a disease or better measuring treatment efficacy compared to traditional measures). In addition, due to potential within-class variation, or inconsistent results amongst a given class of digital devices (e.g., wrist-worn activity trackers), even similar devices would likely need individual FDA approval. In a study of apps and wearables, for example, the three wrist-worn wearables (FitBit Flex, Nike Fuelband, and Jawbone UP24) demonstrated marked variation in the number of steps counted. As a result, the focus of the FDA review, in addition to the device, would be on the digital biomarker itself.
Infrastructure must bridge existing data silos and support the storage, analysis, and usage of digital biomarkers.
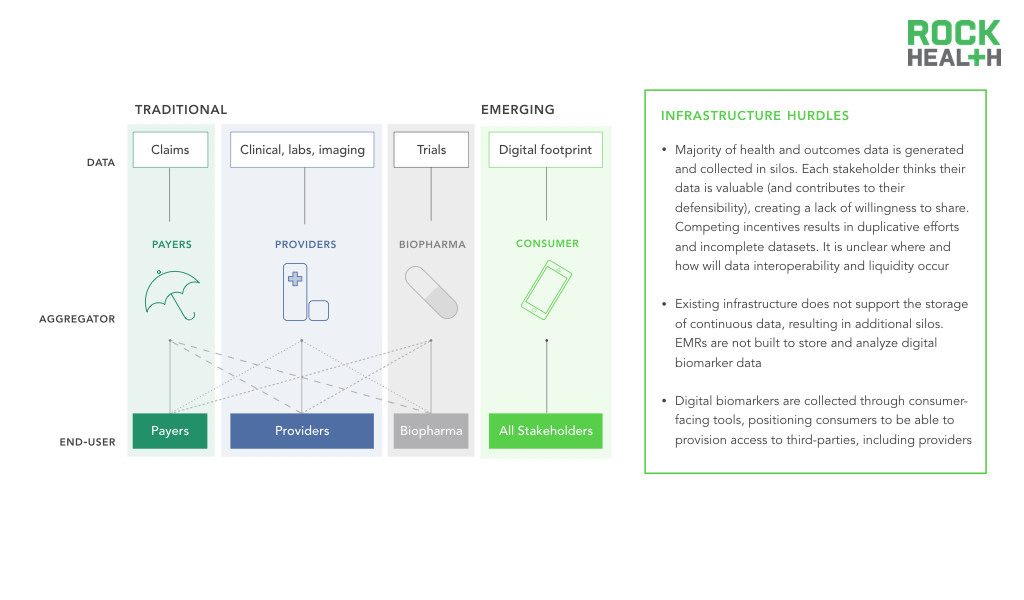
While an interest in collecting digital health data may exist, a clear infrastructure challenge remains for how this data is collected and where it should be stored. Current EMRs are not structured to store large amounts of continuous data, nor are they designed to process analytics in real-time.
Traditionally, healthcare data collection primarily occurs when patients present themselves at physicians’ offices or hospitals. The data is collected and controlled by individual entities, and as a result, data silos arise and limit data liquidity, or the ability of data to flow throughout the healthcare system. Moreover, the data is often unstructured or tagged in a manner that makes exchange across platforms challenging. Standardization of how data is stored, labeled, and tagged allows for more than just the ability to process exchanged information—it provides context around each data point. For instance, in HealthKit, individuals enter glucose readings without denoting if they were taken after fasting versus after a meal. With the proper coding systems, these distinctions can be more readily made and used by clinicians. Existing infrastructure also does not support the storage of continuous data, resulting in additional silos. As consumers generate and collect this data, infrastructure needs to evolve to support its storage and integration with existing data silos.
Uncertainties around stakeholder incentives and workflow integration are key hurdles to clinical adoption of digital biomarkers.
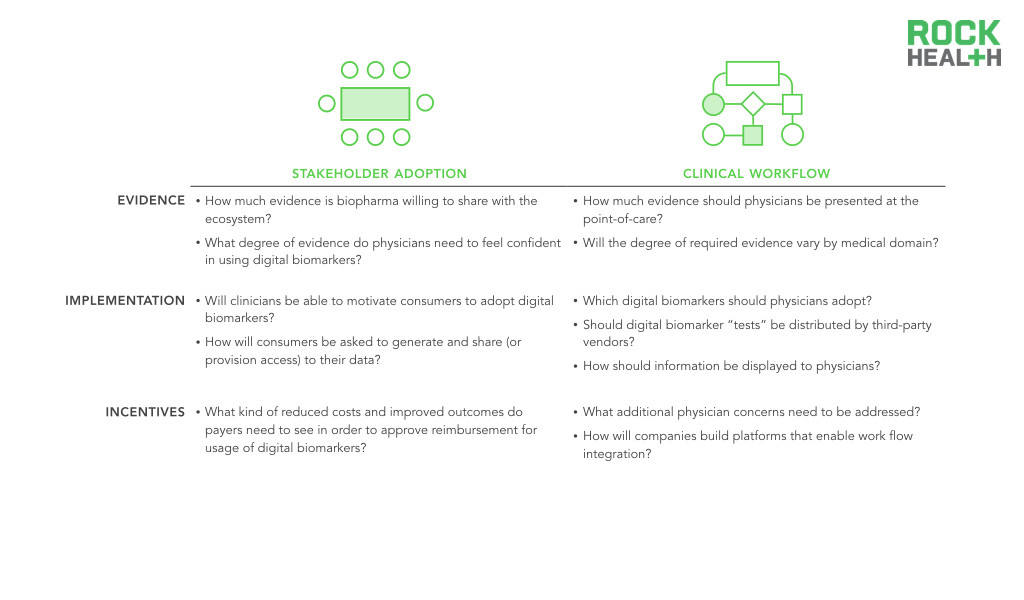
At the end of the day, physicians want to do what’s best for their patients. If you can clearly demonstrate outcomes with evidence, you’ll have adoption into clinical workflow.
Acknowledgements
This report would not have been possible with the help of a number of industry partners and startup entrepreneurs who have graciously shared their expertise.
Thanks to Russ Altman, Stephen Friend, Malay Gandhi, Adam Jones, Jessie Juusola, Deb Kilpatrick, Christine Lemke, Craig Lipset, Philip Ma, Nickolas Mark, Sai Moturu, Lonny Northrup, Rachel Sha, Eric Topol, Mark Weideman, Janet Widmann, and Elizabeth Wu for their time and insights.
And a special shout out to our incredible marketing communications team, Mollie McDowell and Lauren White!