How should the FDA approach the regulation of AI and machine learning in healthcare?
Rock Health and Evidation convened experts to explore key questions—and we want to know what you think.
TL;DR
After a day of dialogue with academically-trained industry experts, we’ve summarized key questions AI/ML technologies create for future FDA regulation. And we need your help to source the answers! We’ll share the learnings with the community—and the FDA—on an ongoing basis.
Send us your feedback and answers to the key questions in the form at the bottom of this page.
Background
Almost weekly we see new headlines proclaiming artificial intelligence and machine learning (AI/ML) will change the world. Many of the clinical applications of AI/ML will be regulated by the Food and Drug Administration (FDA), for instance, as software as a medical device, medical imaging, or clinical decision support tools. Our recent white paper demystifies some of the hype—and includes our take on how to classify the algorithms underlying AI use cases. Despite the extensive research behind that publication, we came away feeling the regulatory implications of this rapidly evolving class of technologies were receiving less than their fair share of the limelight.
To address this, on March 15, 2018, we joined forces with our portfolio company Evidation to convene an AI/ML working group of 18 experienced leaders across healthcare, representing the regulatory, startup, enterprise, and academic sectors. The FDA’s Associate Director for Digital Health, Bakul Patel, graciously observed the day-long discussion.
Rather than tackle the topic through the lens of regulatory burden or rule-making and compliance, the group focused on identifying emerging themes that would help the FDA meet its mandate while also creating a “level playing field” for industry. A clear, core set of issues and questions came to light and are outlined below. These issues reflect a starting point for further exploration of some of the challenges both innovators and the FDA will face as they work together to bring validated, high-value products to the market that leverage machine learning models.
1. When seeking ground truth data (see definition in the graphic below), how should entrepreneurs navigate the following challenges?:
- Consensus ground truth data (also referred to as “benchmark data”) is often not available “off the shelf” or reflects inherent variability in real-world clinical practice
- Uncertainty exists about what may or may not be “acceptable” to regulators as ground truth1
Additional questions to consider:
- What is the role for industry and/or academia in generating “acceptable” ground truth baseline data sets for widely applicable AI/ML problem domains?
- Companies must choose from among different data sources and collection methods. What guidelines or best practices can be established surrounding retrospective data/studies versus prospective data/studies when developing regulated AI/ML models?
- What guidance could regulators provide regarding the forms of ground truth baseline data acceptable across general use cases? Specific scenarios?
- How should the FDA approach scenarios in which ground truth may not be easy to generate or easily accessible?
- For example, human pathologists may not be able to accurately quantify the simultaneous presence of multiple immunohistochemistry (IHC) assays in a single piece of tissue as well as a machine can; how should “ground truth” be established in situations where humans may not be able to provide an accurate assessment?
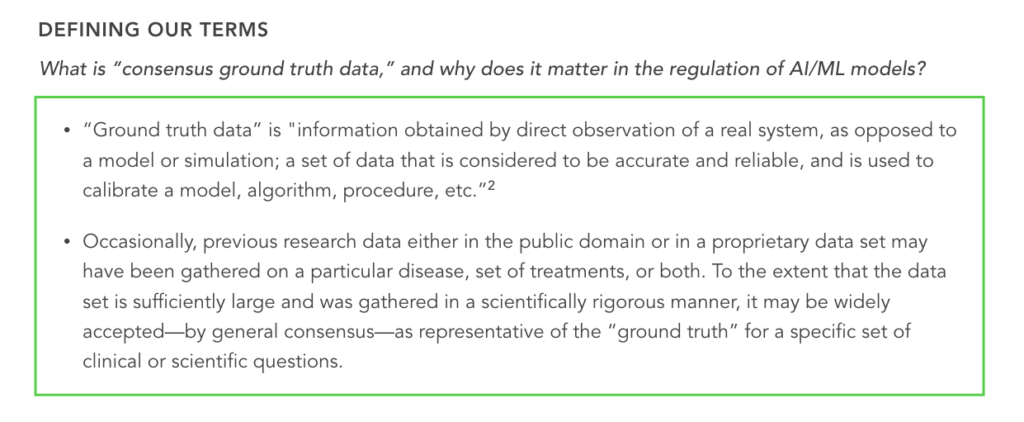
Ground truth is required to train AI/ML models and to demonstrate their effectiveness. When a consensus ground truth dataset isn’t available, it must be collected. And because there are many possible ways to collect or generate ground truth data, this represents a gray area in which clarification could be helpful.
- For example, to develop an AI/ML model that improves patient outcomes through adherence to a clinical guideline, the model builder would need a data set that contains data on patient outcomes under current clinical guidelines. However, clinical guidelines often do not have a clear ground truth because they may not be based on large-scale validation studies. The same problem exists for wellness interventions, which are even less likely to have a ground truth.
A related but separate question arises concerning what constitutes an acceptable “source” for ground truth. For example, it makes intuitive sense that any new AI/ML model should rely on ground truth data representative of the current “standard of care.” However, “standard of care” data is based on the standard practice of clinicians—and in important scenarios, physician practice is subjectively variable. Therefore, any models trained from standard practice data will reflect any embedded errors or may be weakened by “noise” introduced due to inherent human variability.
How should the FDA approach this potential issue?
- For example, the FDA may expect some companies to train their models on clinician-labeled data, but that data would then potentially embed the practice variability they are intended to combat.
2. Because data is used to train AI/ML models, it is the “active ingredient” in the same way a molecule is an active ingredient within a drug. How should industry and regulators approach issues surrounding data? Should standards for data, transparency of the data used to train AI/ML models, and/or reproducibility of models on similar data be considered?
Additional questions to consider:
- How does the intended use of the product (i.e., the AI/ML model) interact with (leveling up or down) the required “quality” of training data sets and how they are generated?
- What best practices for separating and/or generating training and test data sets should there be?
- To what extent should data (either testing and/or training) rely on publicly available sources?
- How should model builders consider the downstream patient implications of algorithm bias (e.g., denying care to certain populations)?
3. What is the appropriate regulatory approach for refitting models to enable them to learn continuously (as opposed to remaining static)?
AI/ML models have the potential to be dynamic models which improve when refitted (i.e., trained on new data). As FDA Commissioner Scott Gottlieb noted in his remarks on April 26, “We expect that AI tools can become even more predictive as additional real world data is fed into these algorithms.” The working group identified a need for guidance on how the process of refitting models should interact with regulation.
Additional questions to consider:
- How does a company decide when to do a refit of the model and how should this be validated? What is the right refitting cadence for various use cases (e.g., every hour, day, six months, annually)? In what contexts should refitting require regulatory approval (or not)?
- What regulations will ensure a company is refitting and shipping models appropriately?
- How do you spot and correct errors such as overfitting?
- What regulatory rules are required around the generation of and potential reuse of holdout data sets?
4. What attributes of an AI/ML healthcare company need to be assessed to determine the quality of its products/services?
This past April, Gottlieb announced the FDA is placing focus on AI/ML within the Digital Health Software Precertification Pilot Program. The Pre-Cert pilot is exploring guidelines to help manufacturers demonstrate a robust culture of quality—including the safety and effectiveness of its processes and software developers—which would enable the FDA to streamline approvals.
Check here to learn more about Rock Health’s and our portfolio company Enzyme’s take on the FDA’s recent working model draft of the Precertification program.
Though the AI/ML working group took place before Gottlieb’s announcement, the group discussed the potential implications of Pre-Cert. We invite the broader community to share feedback on specific considerations that should be incorporated into the Pre-Cert program to address the needs and opportunities of AI/ML driven solutions. Feedback may touch upon:3
- Quality management systems and infrastructure
- For example: To what extent should software versioning procedures be applied to training data packages (alongside the software of the AI/ML model itself)?
- Robustness of pre-market model testing for safety/basic efficacy
- Post-market monitoring
- Validation/verification protocols
- Anomaly detection systems
- Identifying adverse events and conducting root cause analyses
We hope the digital health community—you!—will engage with these questions. We are eager to hear your feedback. Here are the next steps:
- Please fill out the form below with proposed answers, comments, or perhaps additional questions.
- We’ll share what we receive with you and the FDA later this year.
Special thanks to our AI/ML healthcare working group participants for sharing their experiences, insights, and feedback which guided this post.
John Axerio-Cilies, PhD, Arterys
Joshua Bloom, PhD, GE Digital
Ian Blumenfeld, PhD, Clover Health
Alison Darcy, PhD, Woebot
Erik Douglas, PhD, CellScope
Bill Evans, Rock Health
Luca Foschini, PhD, Evidation
Leo Grady, PhD, HeartFlow
Liam Kaufman, WinterLight Labs
Christine Lemke, Evidation
Janine Morris, Lilly
Katie Planey, PhD, Mantra Bio
Ryan Quan, Omada Health
Sarah Smith, Bodyport
Megan Zweig, Rock Health
Some working group participants preferred to remain anonymous.
1Experts in the working group noted there may be reason to distinguish between the use of benchmark data in training versus testing the models. We invite the community to consider and respond to the questions in this section with both scenarios in mind.
2Ground truth [Def 3]. In Oxford Living Dictionaries, Retrieved May 17, 2018, from https://en.oxforddictionaries.com/definition/us/ground_truth. At least one academically trained researcher from this group suggested that this definition may be too narrow noting that, among other things, ground truth may originate as output from another validated model.
3Many of these are considerations are already incorporated into the FDA approval process.
Want to share feedback? Fill out the form below.