From buzzword to business case: Constructing AI use cases for pharma
Across pharma (well, let’s face it, across healthcare…and every other industry) everyone’s talking about artificial intelligence, or AI. There are plenty of recent articles describing AI use cases that will transform the pharma value chain, and there are more than 130 digital health startups in the U.S. alone selling AI-enabled tools for pharma tasks.1 News headlines tout relationships between drug makers and Big Tech AI leaders like OpenAI, Microsoft, Amazon, and Google.
With all the noise around AI’s potential, it can be hard to identify the concrete ways AI could improve jobs-to-be-done at your organization and determine if there’s a business case for implementation. To support pharma leaders in moving from buzz to a plan, we’ve developed a two-phase approach that helps leaders build specific AI use cases that raise the right questions to support business decisions.
Phase 1: Construct an AI use case
We’ve all heard broad statements about the potential for AI transformation (e.g., “AI will disrupt clinical trials,” “AI will transform pharma advertising”), but they don’t get to the mechanics of how the technology impacts specific jobs across the pharma life cycle. It’s helpful to construct use cases that define how AI acts on your team’s job(s) to be done. The anatomy of an AI use case includes:
- The job that AI will support (what are you solving?)
- The action that the algorithm will do to change the job (how will you solve it?)
- The impact the action will have on the job (how will the workflow and team have to change?)
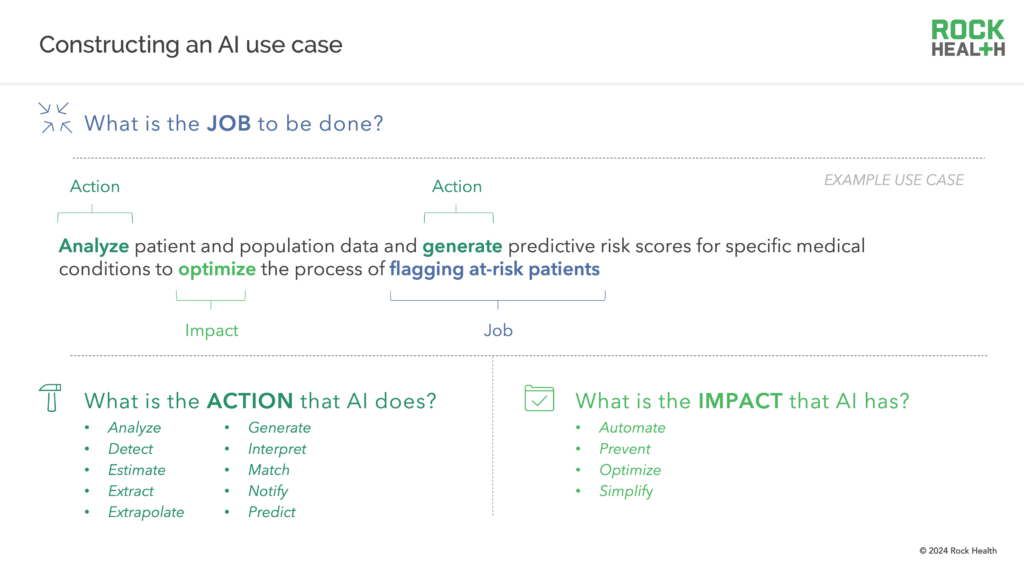
Almost all AI tools act on very specific jobs in a department, team, or function. For example, instead of “AI for pharma advertising,” AI jobs include generating content for marketing materials, reviewing ads for regulatory and compliance risk, or analyzing marketing mix. “AI for clinical trials” tackle jobs like drafting protocols, selecting trial sites, or matching patients to trials. Sometimes AI tools are bundled together into platforms or product suites, but evaluation should happen at the job level.
Actions that AI can complete vary, and options will grow as AI capabilities evolve. Actions like sort (molecular compounds), match (patients to trial eligibility), and score (quality measures at manufacturing sites) are probably already familiar and stem from machine learning models. Generative AI is introducing new action possibilities like enhance (medical and scientific imaging), create (custom field sales content), and chat (with consumers). New types of actions bring new considerations for risk and regulatory oversight. Keeping track of the menu of AI actions you’re implementing will be even more important as multimodal AI (software in which multiple AI algorithms interact) becomes more common.
The impact statement plugs the job and action back into the broader process, and signals how workflow or team roles might change in light of AI’s actions. If AI automates a process, team structure could change; if the process is streamlined or optimized, staff workloads would reduce and could evolve to include reviewing an AI output.
To demonstrate this approach, let’s construct a use case within the context of clinical trials.
1. Pick a job. Consider the job of refining eligibility criteria for clinical trials, a process with multiple iterations. If the criteria is too niche, not enough participants will enroll in the trial; if the criteria is too broad, trials suffer from increased variability and higher management costs.
2. Distill what actions the AI would do. To refine eligibility criteria, AI would:
- Estimate the number of patients who are eligible to participate in a trial based on certain criteria
- Simulate trial protocol, evaluating trial enrollment and completion rates as well as features such as hazard ratios
- Calculate adjustments to the criteria to increase likelihood of study success
3. Spell out the impact. By taking these actions, AI tools optimize the process. Investigators will still need to review the criteria, but their workload within the protocol drafting process will be reduced. Downstream benefits include higher likelihood of trial success and ultimately lower drug development costs.
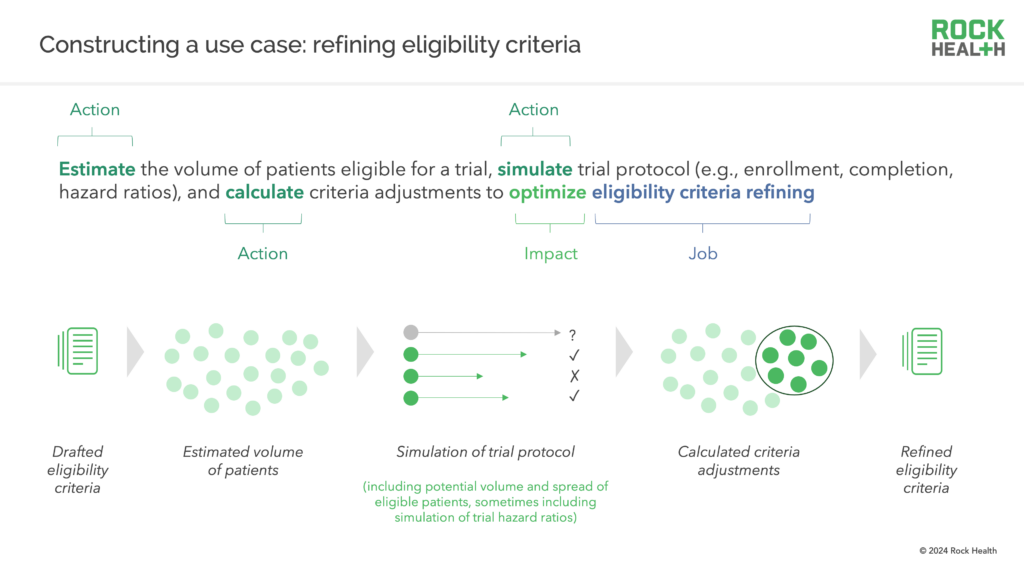
Phase 2: Ask the right questions
By understanding the impact of AI on specific jobs, you can get to more granular questions about how to execute on a use case and whether it makes sense for your business.
How do you execute?
- What data do you need?
- What algorithm set should you use (custom, tuned, off-the-shelf)?
- Do you need a partner? What type (enterprise, startup)?
What will change in your process?
- Given the job and the impact, how does the workflow change?
- Where is the human in the loop?
- What is the impact on your team structure?
- What do you need to train your team on?
- What level of risk and regulatory burden will you assume?
Is there a business case?
- What is the time horizon for realized benefits?
- Is the outcome worth the investment?
Looking at the question of partner selection, let’s say you’ve developed the use case of using AI to generate promising molecular structures to optimize the process of de novo drug discovery. This job starts with open-source datasets, so you need to get those externally. The AI actions of generating molecular structures from that data could be done internally if your team has that capability, or could be done in partnership with a digital health player with that capability. Optimizing the process assumes you can dedicate less team resources to it.
Getting started
Constructing clear use cases is a first step to help get past the AI buzz and move to business decisions.
Once you have an inventory of use cases you intend to pursue across your organization, that opens up a new set of questions around your organization-wide strategies to build/buy/partner for AI capabilities.
If your pharma or healthcare organization is looking to better understand, prioritize, and operationalize AI use cases, reach out to advisory@rockhealth.com.
Get in touch with the venture team at Rock Health Capital.
Join us in building a more equitable future at RockHealth.org.
And last but not least, stay plugged into the Rock Health community and all things digital health with the Rock Weekly.