Demystifying AI and machine learning in healthcare
We’re excited to announce the release of our report, Demystifying AI and Machine Learning in Healthcare. We wrote this report because we were tired of AI in healthcare cover stories with pictures of robots, of debates focusing on the semantics of the space instead of the substance, and of exaggerated claims that AI is either the savior or destroyer of healthcare as we know it. As CNBC’s Chrissy Farr put it at the 2017 Rock Health Summit, “It’s tough to distinguish between which companies are actually doing AI/ML and which ones have a spreadsheet in Excel.”
Uncertainty has the potential to obscure or even hold back legitimate, valuable innovation. With a healthcare system in desperate need of transformation, we wanted to equip ourselves—and others—with information and tools that would help us navigate this complex terrain. Our ambition with this report is to separate hype from reality: to offer what we learned as we investigated where AI/ML is truly transforming the business of healthcare and the practice of medicine; where and why investors are placing particular bets; and how the industry can successfully navigate risks and barriers to adoption and scale.
In this blog post—the first of a series—we offer a snapshot of the full report. We’ll kick it off by exploring the first of three key challenges inhibiting innovation, investment, and partnership decisions on AI/ML in healthcare:
A lack of understanding of underlying AI/ML algorithms both makes it easy for companies to inflate claims and also makes it difficult for potential investors, partners, and customers to identify true breakthrough innovation.
So what exactly is AI/ML? (Hint: It’s not magic—just math.)
In the course of researching this report, we were not able to identify a single, agreed-upon definition for “AI/ML”—nor could we gain consensus among either the academically-trained industry practitioners or leading academic researchers we spoke with.
To be clear, there is no particular technology or product specifically called “AI.” Instead, AI/ML refers to the human-like capabilities of specific mathematical algorithms processed by computers. It refers to software applications that can learn—the vast majority of which are machine learning and deep learning algorithms.
The debates over what should be characterized as AI/ML will continue and hopefully yield more awesome tweets. However, it is not feasible (or necessary) to align the entire industry around a single standard definition. Instead, we focus on business value and examine how computer algorithms that learn from data can be used to reach business objectives, such as improving the accuracy of a diagnosis, matching patients to a clinical trial, organizing patients into risk groups, and managing a patient’s depression.
Offering a classification system for underlying AI/ML algorithms
Many different algorithms are capable of consuming data and then generating either an accurate prediction or classification. We took a look under the hood to examine the classes of tools that computer scientists are using to get computers to perform the magic of “data in, prediction out.” And to help entrepreneurs, investors, and enterprise healthcare leaders pragmatically assess the potential utility of AI/ML proposals in their business, we classify predictive algorithms1 into three categories along a spectrum:
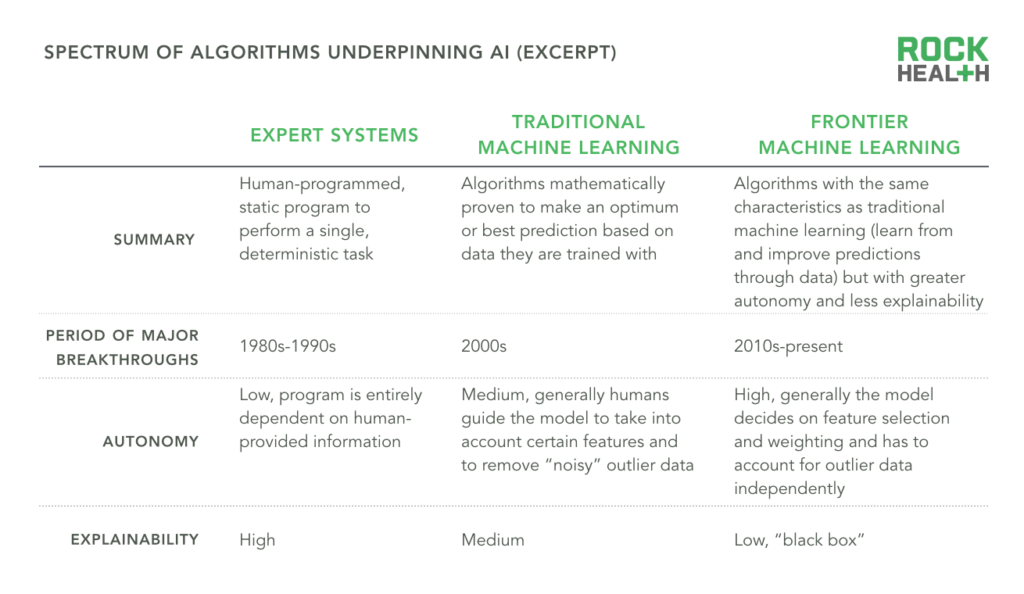
These three categories—expert systems, traditional machine learning, and frontier machine learning—are organized along this spectrum according to two distinguishing attributes:
- Their autonomy as assessed by the degree of human guidance they require to function
- Their explainability—meaning the degree to which humans can examine how an algorithm is coming to a particular prediction or output2
These attributes are inversely related: More autonomous and fine-tuned algorithms require less human guidance; however, it’s more difficult to understand what the computer is doing and why.
Don’t assume “moving to the right” on the spectrum is optimal. More advanced algorithms aren’t always better. Most companies should be assessing and using a variety of techniques. For example, Amino, a San Francisco-based company with an online platform that provides healthcare provider recommendations, cost transparency, and appointment booking, is constantly testing algorithms to find the optimal mix of techniques. They recently tested deep learning algorithms to surface trends on physician specialties and evaluated their technique against two guiding questions:
- What degree of accuracy is necessary to make the product successful?
- What is the incremental improvement from using a more expensive, sophisticated method? Is a simpler technique available?
Against this framework, they decided the added specificity from the deep learning techniques was not worth the added cost in terms of the development time and computing resources needed. Every company using AI/ML should demonstrate an iterative, flexible, yet rigorous mindset in which they seek a desired level of predictive power using the simplest, most affordable techniques available. Investors, enterprise leaders, and others evaluating AI/ML-powered startups can use the Spectrum of Algorithms to guide conversations about the techniques each startup is using, and the utility and intent of using those particular algorithms.
For the complete Spectrum of Algorithms Underpinning AI, with the strengths, limitations, and primary use cases of each algorithm class, read the full report.
This is rapidly evolving terrain—join our ongoing conversation
We hope this is just the beginning of an ongoing dialogue—our priority is to educate and support the digital health community in making smarter business decisions that drive better healthcare. We look forward to hearing your thoughts on which AI/ML-powered use cases excite you—and which ones don’t. We hope you’ll ask us the questions you’re still seeking answers to and help us build out our 2018 research agenda in this space. Send your ideas and questions to research@rockhealth.com. As always, we’re looking to invest in early-stage startups successfully leveraging AI/ML to meaningfully improve healthcare. If that sounds like you, send us your business plan.
Finally, we are indebted to the numerous entrepreneurs, enterprise leaders, statisticians, computer scientists, investors, and others who offered us the advice, feedback, and support which made this report a reality. Thank you!
1This classification is based on historical development of the field and not the mathematical or scientific underpinnings of the algorithms.
2“Explainability” refers to the degree to which the internal structure of the model can be examined to better understand the factors (variables) which most influenced the prediction (output) generated by the model. A predictive model with high explainability for heart attacks, for example, would provide both a prediction of the future probability of a heart attack and also show the degree to which high blood pressure, cholesterol levels, and age (or other factors) contributed to the prediction of a future heart attack. A model with low explainability would only provide a probability for a heart attack with no easy way to see which variables contributed to the prediction.